What is collective intelligence?
At its simplest, ‘collective intelligence’ can be understood as the enhanced capacity that is created when people work together, often with the help of technology, to mobilise a wider range of information, ideas and insights. Collective intelligence (CI) emerges when these contributions are combined to become more than the sum of their parts for purposes ranging from learning and innovation to decision-making.
It has been around for a long time, but the rise of new technologies that connect more and more individuals over greater distances to share knowledge and skills has transformed what can be achieved through CI. In the 19th century, it took almost 70 years to crowdsource the 400,000 words that made up the complete first edition of the Oxford English Dictionary. A modern-day equivalent, Wikipedia, receives 1.8 edits per second and sees more than 6 million new pages created per month.
Figure 2
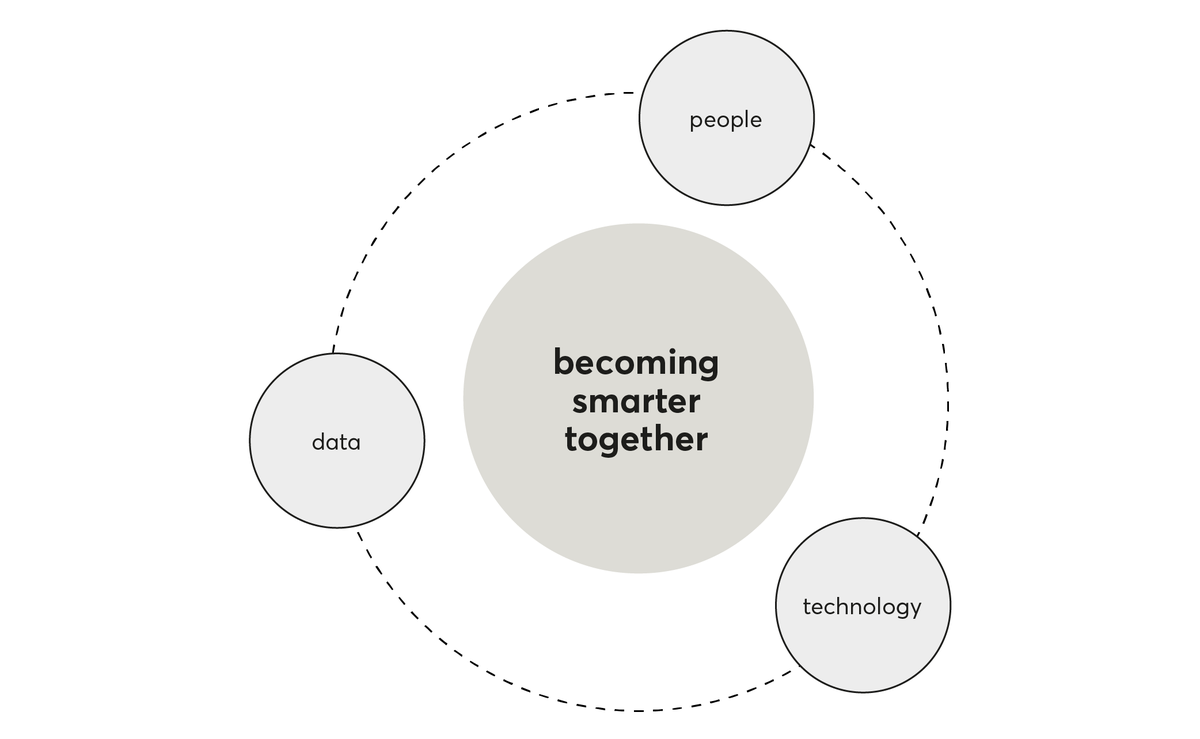
Figure 2 from Nesta's Collective Intelligence Design Playbook
CI covers a wide range of participatory methods, including crowdsourcing, open innovation, prediction markets, citizen science and deliberative democracy. Some of them rely on competition, while others are built on co-operation; some create a sense of community and teamwork, while others operate on the basis of aggregating individual contributions or microtasks. Academic research on CI is equally varied and draws on many different disciplines, including social science, behavioural psychology, management studies and computer science.
CI offers at least four distinct opportunities to help decision-makers and communities solve complex problems:
● Understand problems: Generate contextualised insights, facts and information on the dynamics of a situation.
● Seek solutions: Find novel approaches or tested solutions from elsewhere, or incentivise innovators to create new ways of tackling a problem.
● Decide and act: Make decisions with, or informed by, collaborative input from a wide range of people and/or relevant experts.
● Learn and adapt: Monitor the implementation of initiatives by involving citizens in generating data, and share knowledge to improve the ability of others.
Figure 3
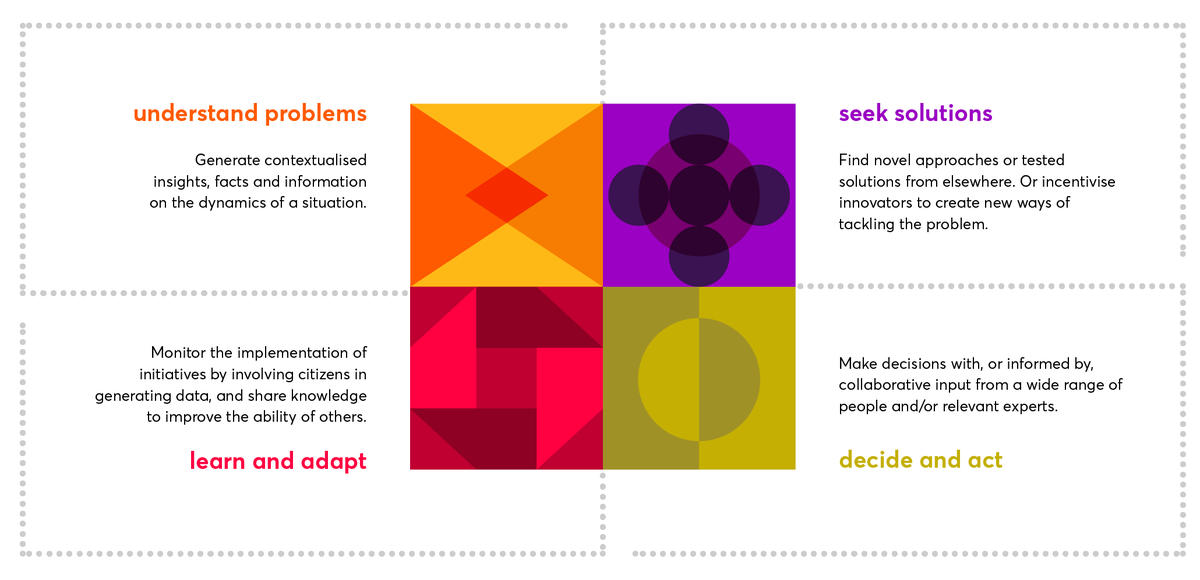
Figure 3 from Nesta's Collective Intelligence Design Playbook
All modern examples of CI rely on bringing together people and/or data or insights in some way. Building on this, the core methods in CI can broadly be described as falling into three categories.
Connecting people with people: The oldest form of CI, bringing people together with other people. It can facilitate distributed information gathering, problem-solving, peer learning and prediction-making. Increasingly, this form of CI combines offline engagement with online contributions, which allows inputs from larger groups of contributors. Methods include peer production, participatory prioritisation, deliberation and open ideation.
CitizenLab is a citizen participation platform that allows local governments to reach out to communicate with the communities they serve. Citizens are invited to submit ideas, prioritise public spending or respond to consultations through an online interface, then the results are sent directly to policymakers. The platform fosters connections between local government and citizens to enable more informed and legitimate decision-making.
Connecting people with data: Brings both people and data together and often involves crowds generating, categorising or filtering unstructured data, such as photos or audio recordings. Some methods offer deeper engagement in processes beyond data gathering, to include participants in the scoping, analysis and evaluation phases of projects. Citizen science, crowdsourcing and crowdmapping are typical such methods.
MapWithAI is part of the digital humanitarian effort, hosted by the Humanitarian OpenStreetMap Team where volunteers create maps in regions where this information is missing or changing due to conflict or environmental disaster. Participants from across the world use images collected by satellites to trace features, such as buildings and roads. These maps help humanitarian agencies to plan missions and response activities that save lives.
Connecting data with data: Brings together multiple and diverse datasets to help generate new and useful insights. These methods increasingly make use of non-conventional data sources generated by people, such as posts on social media, mobile phone geolocation and sensor data. Data collaboratives, open-source repositories and open application programming interfaces (APIs) are some of the methods that are typically used in these data-driven CI projects.
Dataminr is an early warning system that helps organisations to plan and co-ordinate their response to crises. It integrates many non-traditional data sources, including updates posted by citizens on social media, to monitor how situations unfold in real time and create summaries, which are sent as alerts. These updates are tailored to the needs of each client, so they contain the information most relevant to helping them plan and make decisions.
Collective intelligence is more important now than ever before
From the climate crisis, to the displacement and migration of human groups, to rising socio‑economic inequality, it can sometimes feel like the 21st century has been defined by the rise of increasingly complex problems. Unlike simple problems,[1] which follow more predictable trajectories and have obvious fixes, making progress on these complex problems requires dealing with uncertainty and multiple unknowns, where there isn’t just one optimal solution.
This makes them ripe candidates for CI, which draws on a combination of data, technology and diverse human skills to address different aspects of uncertainty.[2]
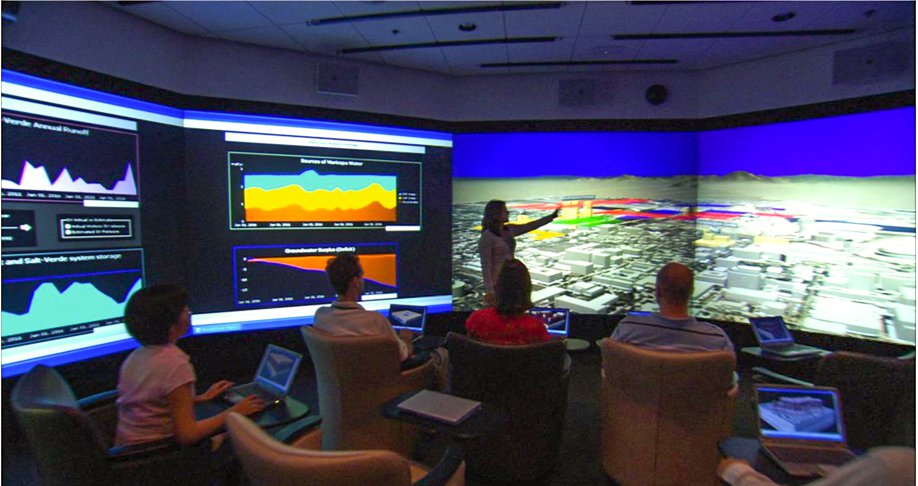
The Decision Theater, Arizona State University
The Decision Theater developed by Arizona State University is a good example of what this CI looks like in practice. It draws on the complementary resources of data, technology and people to create an interactive simulation experience that supports decision-makers to navigate complex problems. To date, it has been used to devise strategies for dealing with problems from land degradation to co-ordinating aid during emergency response. A combination of big data analysis and high-performance computing is used to create detailed models of public policy scenarios. The models are then translated into interactive data visualisations, which are projected onto multiple screens to help teams of decision-makers explore the impact of intervening at various points. Decisions are ultimately made through group deliberation about competing values, trade-offs and priorities, but it is the technology that enables the decision‑makers to engage with the complexity of the problem.
[1] According to Dave Snowden’s framework for problem-solving, problems fall into four distinct categories: simple, complex, complicated and chaotic.
[2] It has been suggested that there are three sources of uncertainty: probability, ambiguity and complexity.