Recommendations
This paper has illustrated that the AI & CI field, while still nascent, holds significant opportunities for using technology to solve some of our most complex social challenges and how we can better involve people in shaping the future trajectory of AI development. However, making the most of this opportunity requires a significant shift in how we think about AI policy, R&D and developing partnerships across different sectors and disciplines.
More than £1 billion has been invested by the UK government into the AI Industrial Strategy, and more than £800 million was raised by AI companies in the first half of 2019. This testifies to the fact that AI is one of the most well-funded areas of research and development. In contrast, we only see a fraction of this funding for CI opportunities. Funds to support the overlap between AI & CI are even more rare. It is thus unsurprising that less than 15 per cent of large companies developing AI technology are actively working to manage risks associated with equity and fairness.
The Nesta fund for CI experiments, delivered in partnership with the Omidyar Foundation, the Wellcome Trust and the Cloudera Foundation, is a rare example of a joint AI & CI fund in the UK. Other countries have shown more foresight. The European Commission plans to launch a new €6 million fund for applied research in this area in 2020. In the US, both the Intelligence Advanced Research Projects Activity and the Defense Advanced Research Projects Agency have funded major projects at the intersection of AI & CI. The multibillion investment in AI research and development in China is also well known, whereas experiments in AI & CI in Chinese cities receive less attention.[1]
The UK has recognised AI as a significant policy priority through the Industrial Strategy Grand Challenges and the AI Sector Deal as well as through the creation of the Office For AI. But there is a risk that setting a trajectory determined by industry priorities rather than viewing AI through a CI lens will lead to public backlash and result in a missed opportunity to use AI & CI to both improve the technology and help enhance collective capacity to solve social problems.
Below we present recommendations for policymakers, funders and researchers on how they can make the most of the AI & CI opportunity.
Policymakers
Put collective intelligence at the core of all AI policy in the United Kingdom.
The UK Government should adapt AI policy to reflect the widest possible collective benefits from AI applications as well as emphasising diversity and broad participation in the development of AI. Specifically:
- The Office for AI, BEIS and DCMS should promote methods where AI enables citizen innovation and collective action to make progress on the Industrial Strategy missions, particularly ageing and mobility, and to ensure the economic benefits of the AI Sector Deal are balanced with social benefit and application of AI in the public interest.
- The AI Council should use emerging AI & CI models and use cases to inform their work on data, narratives and skills of AI and to reframe the UK’s AI opportunity as one that is focused on inclusion and augmenting human intelligence to solve social challenges.
- The upcoming National Data Strategy consultation is an opportunity to use participatory methods to shape future data policy, and to ensure that future AI & CI initiatives are supported by robust data infrastructure and practices.
- The Centre for Data Ethics and Innovation should use its planned State of the Nation report to highlight AI & CI practices and commission a feasibility study for different AI & CI models in the UK public sector context as part of its future work programme.
- The Office for AI and Government Digital Service should update their guidance on using AI in the public sector to include specifications for AI & CI. All procurement of AI tools by central government departments should require vendors to demonstrate CI principles or methods in the development and implementation of tools. The expected guidance on Social value in government procurement should reflect this commitment.
Create testbeds for experimentation to accelerate learning.
So far, experimentation in AI & CI has been ad hoc. Creating dedicated regional or sectoral testbeds allows for experimentation in real-world settings. This could help stimulate private and public sector collaboration and accelerate learning about best practice in AI & CI for public benefit.
Both local and central government should more clearly exploit the opportunities in using AI & CI to increase the quality and scale of existing methods for involving the public in developing policy and delivering public services. While this applies to most parts of public services, we see a specific opportunity for innovation in three areas:
- Digital democracy: Ensure that existing investment in new participatory democracy processes such as the local government citizen assemblies is given an opportunity to create impact by incorporating AI to make better use of citizen’s contributions.
- Environment, energy and climate: The climate crisis and reducing energy demands are two significant challenges where AI could amplify the impact of collective action. Existing citizen science initiatives on air quality, pollution and biodiversity should be integrated into DEFRA policies. Using AI within these projects could help citizen-generated data to achieve maximum impact. Alongside this, Ofgem should work with citizens and industry to explore AI models of different scenarios for future decarbonisation.
- Healthcare and wellbeing: There is a growing need to develop a sophisticated understanding of the socioeconomic determinants of health and to empower citizens to take action to improve their mental and physical wellbeing. NHSx and the NHS AI Laboratory should work with patient and community groups to identify opportunities for developing AI that combines multimodal analysis of health data and non-traditional data sources like open data on GP prescriptions, with lived experience.
Funders
The first major funder to put £10 million into this field will make a lasting impact on the future trajectory for AI and create new opportunities for stimulating economic growth as well as more responsible and democratic AI development.
Launch a new dedicated funding programme for AI & CI research and development.
There are currently no large-scale funding opportunities in the UK for AI & CI research and development. This gap could be filled by UKRI and foundations dedicated to solving societal challenges, like the Wellcome Trust, Open Society Foundations and Luminate.
Public funders should also focus on integrating AI & CI opportunities into existing AI funding programmes and commit to supporting a significant proportion (at least 20 per cent) of all AI projects that explicitly focus on the involvement of people and/or societal impact. Foundations have a specific opportunity to shift current AI4Good funding by foundations towards a clearer focus on how AI can empower collectives and ensure long-term societal impact.
Invest in new partnerships and governance models for AI & CI experiments.
The relative disconnect between the fields of AI and CI along with failures of systems-level co-ordination and governance threaten the success of AI & CI projects.
- Funders need to incentivise interdisciplinary collaboration between the fields of AI and CI by making funding criteria contingent on a partnership approach. The Office for Civil Society, the Knowledge Transfer Network and the Catapults should help to broker public–private partnerships across different sectors.
- Independent organisations like the Ada Lovelace Institute and the Open Data Institute, as well as the Office for AI, should provide guidance on new models of data trusts and oversee public auditing of AI used in the public sector. This will help to ensure the responsible development of AI in the public interest.
Research and practitioners
Looking beyond the research questions raised by the report, there are a number of systemic interventions that are necessary to ensure the continued growth of the field. The academic institutions and technology companies that change the emphasis of their AI research and development programmes towards collective intelligence and applications of AI in the public interest will have ‘first mover’ advantage and be recognised as global leaders in this emerging field.
Build a new interdisciplinary field and link to real-world practice.
The field of AI & CI covers a broad range of subdisciplines in which the UK research community is recognised as one of the global leaders, such as AI and citizen science. However, the UK has no academic institution or discipline dedicated specifically to understanding the field of AI & CI, which limits our understanding of current and future opportunities.
- Institutions working across the relevant fields, such as UCL, the Oxford Internet Institute and the Alan Turing Institute, could advance this agenda through dedicated research programmes. This could build on international lessons from similar initiatives such as the MIT Centre for Collective Intelligence in the US and the UM6P School of Collective Intelligence in Morocco.
- Progress in the field could be further advanced through the creation of a dedicated international academic journal which strengthens the links between different research fields and practice-based CI.
Accelerate progress on AI & CI research by committing to open science and evaluation.
Currently, significant resources are being wasted and efforts duplicated due to the lack of access to existing knowledge and solutions, such as data and software. Existing AI & CI projects do not put enough resources into evaluation and sharing of lessons learnt, which risks the repetition of mistakes within the projects and by others in the field. All researchers and practitioners working in AI & CI should:
- Apply the FAIR principles to data management, and follow emerging guidance on sharing data and code to encourage transparency and ensure reproducibility.
- Openly publish on feasibility studies and costs associated with AI & CI solutions.
- Develop new criteria for AI & CI design and new benchmarks to measure performance and impact evaluation (see below).
___
Practice – getting the design of AI & CI projects right
The field can only evolve through more organisations experimenting with different models of AI & CI and the opportunity to deliver novel solutions to real-world challenges. However, beyond ‘just’ calling for more experimentation by practitioners with these new methods, we put forward the following criteria that should be considered in any AI & CI project.
These questions are intended to guide more in-depth consideration of the integration of AI and CI. Practitioners should use them to help plan their projects and as a starting point for project evaluation. The first and last question should always be: Does/did this project really need AI?[2]
- The problem: What issue are you working on? What other methods exist to answer the same question(s)? What are the limitations of current approaches, and can these be addressed by the integration of AI & CI?
- Performance of AI: What is the algorithm optimising for? What existing metrics can be used to continuously monitor AI performance, and what additional criteria are needed to measure the impact on CI initiatives?
- Social acceptance of AI: Are the participants in the project aware of the use of AI? Will they be consulted about its deployment? Do the participants have a choice to opt out of AI-enabled functions?
- Transparency of the algorithm: To what extent is the model interpretable? Is the training data available? Is it possible to verify biases and track model or data set drift?
- Ability to achieve collective goals: Does AI enhance the CI initiative’s progress towards understanding problems, seeking solutions, making decisions or collective learning? What baseline can this improvement be measured against?
- Quality of participation: Is AI enhancing the quality of participation? How does AI change the participatory process (e.g. facilitation, reduction of bias, surfacing new information)?
- Level of interaction between the crowd and AI: What different models of AI and group interaction are most relevant to this project? How serious is the context? What are the risks associated with more/less autonomous implementation of AI?
- Resources and sustainability: What costs are associated with the project in the short and long terms? How much money will be required for data acquisition, storage and analysis, technical support and community engagement? What is the environmental impact?
- Partnerships: Does the project require partnerships between the non-profit, public and private sectors? What governance processes will be followed? What is the value proposition for each side?
Explore 20 projects that bring Artificial Intelligence and Collective Intelligence together
Artificial intelligence and collective intelligence case studies
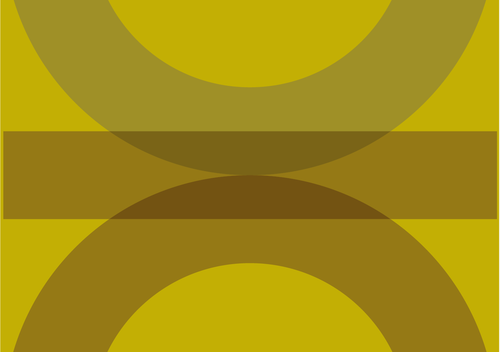
[1] The Alibaba City Brain technology, which has been deployed in Shanghai, Suzhou and Hangzhou, uses AI powered by citizen-generated data to make forecasts and improve public service provision (while also raising concerns about the ethics of deploying, for example, facial recognition technology).
[2] For those new to CI, we recommend Nesta’s Collective Intelligence Design Playbook, which features design questions and resources for project development from problem definition to identification of real-world impacts.