Design choices at the heart of AI for CI
Too often, debates about the development and use of AI are framed in terms of economic gains without due consideration of its wider impacts on values, such as equality and environmental justice. There are a number of other significant tensions that are important to consider before venturing into the world of AI & CI design. Very few of the examples we found had publicly available information about the costs and trade-offs they had considered during the design of their projects, making it difficult for others to learn from their experience. Below, we explore six design challenges at the heart of AI & CI projects that innovators need to consider when applying human and machine intelligence to help solve social problems.
Optimising the process: project efficiency versus participant experience
Several examples of AI integration in CI projects use data that had been previously classified (labelled) by volunteers to train machine models. These AI models are then deployed within the project to perform the same tasks alongside volunteers. The Cochrane Crowd project, which categorises clinical reviews, and the Snapshot Wisconsin project, which analyses camera-trap images on the Zooniverse platform, have both introduced machine classifiers in this way to identify the simplest cases among their large datasets, leaving the more unusual tasks to their volunteers.
Although this approach helps to advance project goals by completing tasks more efficiently, evaluations of both projects found that it risks disincentivising volunteers by making their tasks too hard and too monotonous. Apart from the potential damage to relationships with volunteer citizen scientists, it may also reduce broader social impacts. These include increased science literacy and behavioural change with regard to environmental issues, which are an important part of CI projects.
One of the great benefits of AI systems is their speed and ability to perform consistently. In contrast, large-scale CI participatory methods can require more time to acknowledge and interpret contrasting values and viewpoints. Projects need to decide on the optimal balance between AI & CI and the different functions they provide depending on the issue at hand. For example, when citizens are brought together to deliberate on contentious or complex issues, as is the case on digital democracy platforms, they may choose to optimise the methods used for transparency and inclusiveness, which may in fact slow down the process. In other contexts, such as anticipating and responding to humanitarian emergencies or disease outbreaks, the high speed of AI methods may be prioritised at the expense of involving a wider array of stakeholders.
Developing tools that are ‘good enough’
In AI development, the primary focus is often on developing the best possible technology by, for example, creating the most accurate prediction model. However, developing something that is just ‘good enough’ can often lead to better uptake and value for money.
Whether choosing between passive or active contributions from the crowd, classical machine-learning or more cutting-edge techniques, you should be guided by the principle of ‘fitness for purpose’. This metric will be more or less focused on accuracy, depending on whether AI is being used as a triage mechanism or to issue assessments in high-stakes scenarios, for example making diagnostic assessments. In reality, gains in accuracy are sometimes minimal in comparison to simple models or even traditional statistics.
Some of the most cutting-edge AI methods, such as deep learning, are developed in laboratory settings or industry contexts, where a detailed understanding of how the algorithm works is less of a priority. CI initiatives that involve or affect members of the public carry a higher risk of widespread impact when things go wrong, for example when projects address complex social issues such as diagnostics in healthcare or management of crisis response. This places a higher burden of responsibility on CI projects to ensure that the AI tools they use are well understood.
Some companies that initially develop models using uninterpretable methods can be forced to discard them in favour of simple machine-learning models to meet the stricter accountability norms imposed by working with the public sector or civil society. One example is NamSor, an AI company working on accurate gender classifications who switched to working with simpler, explainable models in order to work with a university partner. Testing new methods in the more realistic and complex virtual environments, such as Project Malmo from Microsoft, and formalising standards of transparency for public sector AI can help to overcome this gap between performance in development and the real world.
Managing the practical costs of running AI & CI projects
Developing CI projects can be costly. Weighing up the ambition to involve the widest group of people and use cutting-edge AI tools will always need to be balanced against the significant costs of doing this. The resource demands will vary depending on the size of the group involved, the depth of engagement and duration.
The integration of AI-data-centric methods adds considerably to these costs through the need to access specialised expertise, additional computational demands and maintain data storage. Some of the costs can be shouldered by private sector tech partners. Examples of this kind of sponsorship include the iNaturalist Computer Vision Challenge, which was supported by Google, and the Open AI Challenges model developed by WeRobotics, which are typically financed by a consortium of public and private sector organisations. Another example is the Chinese AI company iCarbonX, which partnered with the peer-to-peer patient network PatientsLikeMe. In 2016, iCarbonX established the Digital Life Alliance, a collaborative data arrangement to accelerate progress on AI systems that can use biological data in combination with the lived experience of patients to support decision‑making in health for patients and medical practitioners alike.
Recent changes to the use of AI in practice, such as better documentation of training data and new tools for detecting dataset drift or describing model limitations, may help smaller organisations navigate the trade-offs of available technology. However, the extra administration required to implement these practices could create a resourcing burden that larger institutions are able to absorb while smaller organisations, charities and community groups struggle. This may mean that few will be able to take advantage of these methods.
Wider societal costs of popular AI methods
Alongside technical challenges and impact on volunteers, any project working at the intersection of AI & CI needs to take into consideration the potential wider societal cost of its use. A common critique of AI is the strain that its development and deployment can place on the right to decent work, environmental resources and the distribution of wealth and benefits in society. This stands at odds with the problems that we turn to technology to solve, such as social inequality and the climate crisis. In fact, some of the latest AI methods are so computationally demanding that the environmental impact of training them has been estimated as equivalent to the lifetime carbon footprint of five average cars.[1]
Studies into the use of AI systems in areas such as criminal justice and social welfare have shown that smart machines can reinforce historical biases and uphold political value systems. CI, when done well, offers a potential counterpoint to this challenge by opening up public problem‑solving to citizens and under-represented groups. Unless these issues are targeted from the outset, there is a risk that participatory methods become a vehicle for consolidating existing power hierarchies.
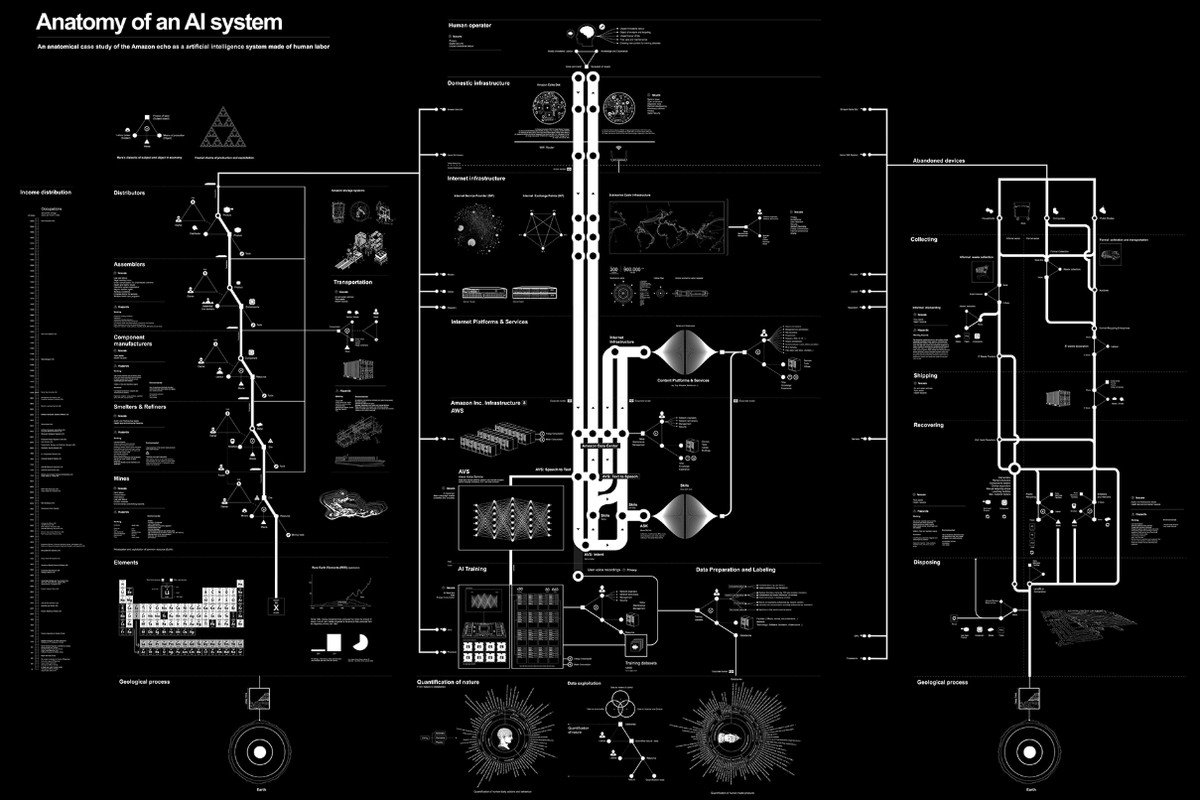
The ‘Anatomy of an AI system’ is a detailed mapping of the crowd labour behind the production of an Amazon Echo, developed by Kate Crawford and Vladan Joler. It raises important questions about the right to fulfilling work and acceptable standards for crowd work, as well as highlighting unsustainable resource demands of the supply chain. Transparency about these different trade-offs can help guide more informed decisions and stimulate public debate about the normative values that should govern the design of solutions to public sector problems.
Open data, open-source tools versus vulnerability to misuse
Open data and software are key enablers for CI initiatives. Opening up datasets and involving more individuals in the development of solutions can help to stimulate innovation by generating more creative ideas or spreading the use of existing solutions to new contexts. Publicly available data can be used to develop entirely new CI projects and open up markets to new providers. Precision agriculture company OneSoil, for example, applies AI to high-quality free and open-source satellite images in order to map field boundaries, crop types and plant health on farmland in near real time to support farmers’ decision‑making. In some cases, particularly when CI is used for participatory democracy, open data and software provides transparency to ensure the verifiability of public processes. As an example, the entire collective decision‑making process for the participatory democracy project vTaiwan is conducted using open-source tools.
However, when data is contributed through passive or active crowdsourcing, it may contain sensitive information that is vulnerable to misuse. If placed in the wrong hands, personally identifiable features of data collected in humanitarian contexts or measurements that reveal private health information might create issues for contributing individuals. The use of passive citizen contributions for AI & CI initiatives in the public sector (e.g. mobile geolocations, conversations on social media, voice calls to public radio) may require a recalibration of the social contract to decide what we deem justifiable in the name of public good and collective benefit.
Cultivating trust and new ways of working with machines
At the heart of AI & CI integration sits the need for a high degree of trust between groups of humans and the machine(s) they work with. In comparison with the resources allocated for enhancing AI systems, relatively little attention has been paid to investigating public attitudes to AI performing a variety of tasks (apart from decision-making). As a result there is a limited understanding of trust and acceptability of using AI in a variety of settings, including the public sector. In How Humans Judge Machines,[2] Cesar Hidalgo and colleagues describe how they use large-scale online experiments to investigate how people assign responsibility depending on whether people or machines are involved in decision-making. The participants in the experiments differentially judged human decision-making by the intention, whereas the decisions made using automated methods were assessed by the harm/impact of the outcome.
When it comes to decision-making as a group, there is an additional layer of complexity around whether individuals feel they carry responsibility for collective decisions. It’s possible to imagine situations where artificial agents help to increase a feeling of shared ownership over consensus decisions, but to take full advantage of these possibilities we need to better understand the variety of individuals’ attitudes to AI. In high-stakes decision-making, for example when automated decision support tools (DSTs) are introduced to guide the work of social workers or judges, they encounter a wide range of reactions from individual team members, such as ignoring or anchoring relying too heavily on the machine’s recommendation[3]. Sometimes automation bias (a tendency to place excessive faith in a machine’s ability) can cause professionals to dismiss their own expertise or use it as a source of validation. We are only at the beginning of unpicking these complex interactions between people and AI and how well they are explained by our existing theories of trust and responsibility
[1] This estimate is based only on the fuel required to run the car over its lifetime, not manufacturing or other costs.
[2] 2020, forthcoming publication
[3] This is known as anchoring.