How human and machine intelligence are different
Like no other technology that has come before it, AI raises questions about the unique value of human intelligence. After all, if computers can do what we do, some of them with even better results, how special is human intelligence?
Such existential questions have been fuelled in part by the quest towards developing what is known as artificial general intelligence, which aims to recreate true human-like intelligence that is flexible and generalises between different tasks, rather than focusing on a narrow set of specialised tasks like most of the AI that we currently see in the world. Unlike human intelligence, these AI methods show little ability to transfer the skills learnt for solving one type of problem into a different context.[1]
The ambition of recreating human intelligence risks losing sight of the potential gains offered by combining complementary aspects of human and computer capabilities.
Figure 4
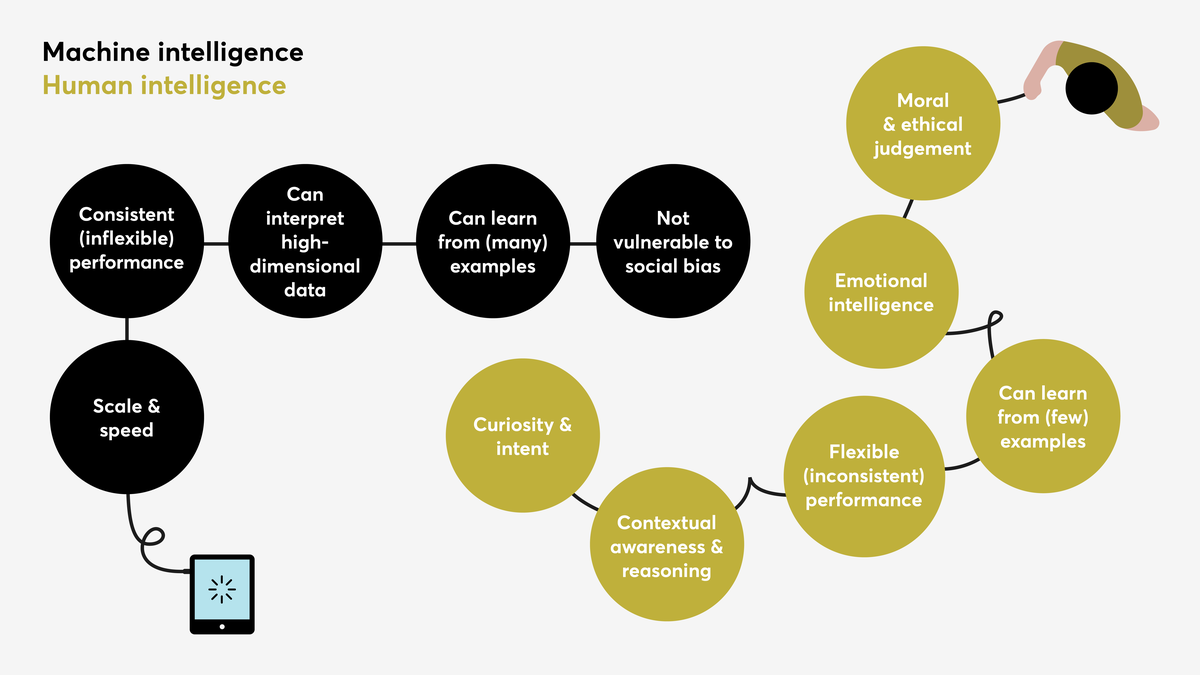
Complementary sources of intelligence for real-world problems
AI models are typically most useful where a ‘ground truth’ is well defined[2] and the data sources that the AI model uses as input do not frequently change. In these situations, the ability of AI to find patterns in huge amounts of data is useful for streamlining decision-making. For example, the medical search engine Epistemonikos uses machine-learning to identify clinical systematic reviews in the academic and policy literature. For many years the definition of a systematic review has been globally agreed by medical practitioners and the formats of studies found in the literature follow a narrow set of templates acknowledged and expected by the sector. These characteristics makes it a perfect candidate for machine-learning because the training datasets are exactly representative of the data that the model will encounter when it is deployed in the real world.[3] Epistemonikos has been used by the Chilean government to increase the efficiency with which policymakers set new health guidelines based on the latest evidence.
However, in the case of many complex real-world problems, like health epidemics and extreme weather events, the dynamics of the situation might lack historical precedent. This issue can result in so-called ‘dataset drift’, which means that the data a given model was trained on is no longer equivalent to the real-world situation in which it is used, so there is no guarantee that the model’s predictions will be accurate. When it comes to high-stakes decision-making, such as co-ordinating disaster response or managing medical emergencies, this inaccuracy can be particularly dangerous.
In these circumstances, the human ability to adapt to new situations, understand context and update knowledge fills in the data gaps of machines. For example, the Early Warning Project draws on crowd forecasting to plug gaps in between its annual update to the statistical models it uses to estimate the risk of genocide across the world. In this context, the collective provides an alternative source of intelligence that can respond to weak signals and unexpected developments that could influence political decisions.
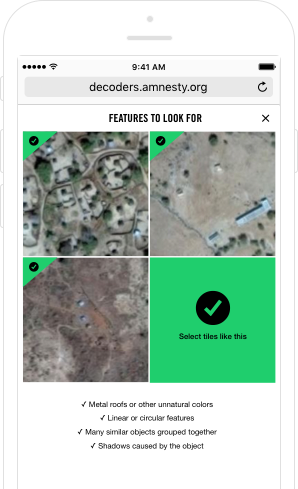
At the collective level, attributes of human intelligence include the ability to tell collective stories as an act of sense-making and learning. For example, Decode Darfur – an initiative run by Amnesty International for their distributed volunteering community, Amnesty Decoders – asked participants to identify areas of destruction in the settlements of Darfur using satellite images. Apart from demonstrating group accuracy that was similar to classification by experts, the project provided an opportunity for the volunteers to discuss what they were seeing as an act of ‘collective articulation of experience’. It is difficult to imagine smart machines taking on this role and yet, when faced with societal challenges, having platforms for discussing and shaping collective values in this way is important.
So far, the well-known Turing test[4] and various specialised benchmarks[5] have been used by industry and academia as measures of the performance of AI systems, but they are primarily measures of relative performance between new AI methods. It is not so easy to develop an absolute intelligence quotient for machines, and the value of distilling intelligence into a single static number is as questionable for AI as it is for human intelligence.
To truly take advantage of 21st-century collective intelligence, where AI is interacting with large groups of people and social systems, we will need to compare and contrast different ways of knowing the world and how best to combine them to solve a given problem. This process requires developing entirely new standards of evaluating performance, including criteria such as the level of human agency, how equally benefits and opportunities are distributed within society and the differential impact of AI on under-represented populations.
[1] Apart from transfer learning paradigms.
[2] More recently, the existence of the ‘ground truth’ principle has been questioned – alongside claims to neutrality – by the data science/AI communities (Emily Denton, Retrospectives Session 3: NeurIPS 2019).
[3] The assumed match between the distributions of training and real-world data is known as the IID principle (independent and identically distributed data).
[4] Proposed by Alan Turing in 1950, the test Can Machines Think? evaluates a machine’s capacity to convince a human that they are interacting with another human.
[5] For example, GLUE battery of language tasks for natural language processing methods: https://gluebenchmark.com/leaderboard