Common pitfalls of AI & CI integration
Despite many promising opportunities in combining AI & CI, it can be easy to make mistakes when bringing together these two often contrasting methodologies. Building on lessons from project failures and challenges overcome by successful initiatives, we outline some examples of how the integration of AI & CI can go wrong and the main design tensions anyone in this field should be aware of.
Big tech hubris
Some of the most common failures of AI integration stem from not adequately considering ongoing human interactions and group behaviour when deploying AI tools. One example is Google Flu Trends, which was hailed as a success of search-query-scraping to predict flu prevalence, before it emerged that it was vulnerable to overfitting[1] and changes in search behaviour. Integrating real-time public health data into the predictions or involving health professionals in the tool’s development might have circumvented some of these shortcomings.
Even well-intentioned projects like the Detox tool developed by Google Jigsaw and Wikipedia, which used crowdsourcing and machine-learning to identify toxic comments, may only be effective for short periods until ‘bad actors’ figure out how to counteract them. The vulnerability to such ‘gaming’ is a common feature of automated methods when they are not updated frequently enough to remain sensitive to dynamic real-world contexts and the potential for negative behaviour from human users. When Microsoft launched a public chatbot called Tay in 2016 as part of their research into conversational AI, they failed to anticipate that some Twitter users would teach the AI agent to make racist comments. Carrying out regular assessments of vulnerabilities and impacts of adversarial actors in any social network is vital to preventing such cases of misuse before they occur or affect too many individuals.
Prioritising marketing over deliberation on platforms
Some social platforms have been criticised for encouraging the spreading of negative comments or amplifying bad behaviour by using algorithms that optimise for features like click-through rates. For example, the popular discussion forum platforms 4chan/8chan have been widely criticised for encouraging, supporting and protecting hate-filled rhetoric, and researchers have claimed that algorithms on Reddit are tuned to incentivise bad behaviour.
Although some of these features are adjustable, changing them still relies on moderator preferences and an active decision by online communities to opt out of default settings. Experiments by CAT Lab, have shown that community behaviours such as regularly reposting community rules can help to counteract some of the negative impacts of AI-enabled platform design by reinforcing social norms.
The importance of design features is similarly highlighted by the deliberation platform Polis, where users contribute their ideas and opinions on a discussion topic. Participants rank each other’s statements but are not able to directly post replies to any of the ideas. The absence of a comment feature was a deliberate choice by the platform’s designers to help promote more open, consensus-driven debate among users. The platform’s visualisations cluster similar opinions to help participants understand where their opinion falls in relation to others’ and how they contribute to the formation of group consensus. Polis is notably used by the Taiwanese government to help identify areas of agreement between different groups as part of their citizen participation project, vTaiwan.
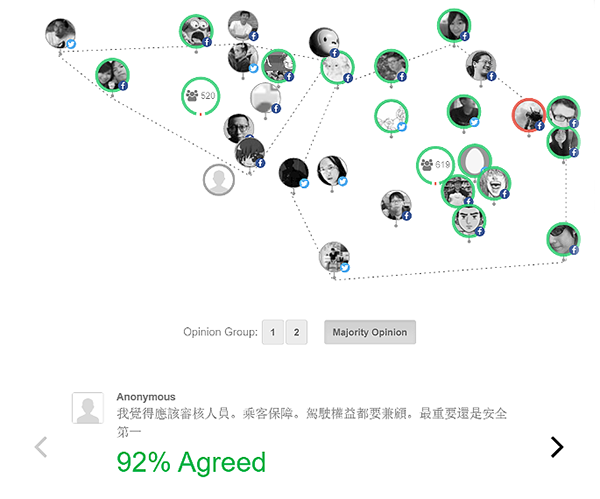
vTaiwan: Screenshot from the Pol.is mass deliberation on ridesharing
Reliance on partnerships between organisations
Data collaboratives fall into the category of CI methods that describe an arrangement to contribute and share data between different parties. For many sectors, the delivery of public services is split between multiple organisations, teams or stakeholder groups so, to make the most of the AI opportunity, these parties need to come together to share data or code. However, the deployment of AI in these contexts can encounter long delays (or complete deadlock) due to clashes in institutional processes and values or difficulty in negotiating responsibilities and resources. For example, the New York City Fire Department, which long promised an enhanced AI-enabled version (Firecast 3.0) of its model for predicting fire risks, has faced many difficulties due to organisational culture.
New pressures on the social contract
There is a thin line between mobilising CI for collective benefit and exploiting users’ data or manipulating crowds. The use of AI as a method of social surveillance (such as through facial recognition technology) has attracted criticism of the Chinese state and led to regulatory bans in parts of the US and Europe. Public sector organisations should navigate these debates with extra care and attention, given that they often lack the resources to develop their own models in-house or to adequately scrutinise commercial AI systems. As organisations in the public sector seek to make the most of the added value offered by AI in the face of continued low public trust in institutions, it will become increasingly important to re-examine and renegotiate the social contract with the communities they serve. For AI-enabled CI to flourish, it is necessary to build automated systems that use responsible data practices and foreground principles of collective benefit.
[1] Overfitting is when models are tuned too precisely to the examples that are used to train them, meaning that they are less able to generalise the new inputs they encounter in the real world.