CI for better AI: The fifth interaction
While the use of AI in CI initiatives is relatively new, CI-based methods have played a significant but often unrecognised role in shaping the development of AI. Below we discuss this evolution and how increased recognition of and investment in CI could help develop better AI.
The hidden story of CI in AI
AI today is much more dependent on groups of people than is often appreciated. Unseen collective labour contributes to the latest advances in AI, especially machine-learning approaches that rely on humans to label the large datasets they use as their training material.[1] Whether these activities are compensated through crowd-work platforms such as Amazon Mechanical Turk and Figure Eight or obtained through internet traffic with tools such as reCAPTCHA, they often remain the untold story behind the success of AI. Concerns about the quality of data labels and the motivations and productivity of crowd workers are the focus of hundreds of papers in the machine-learning research community.
More recently, companies have started to enable industry and researchers to outsource the management of crowd workers. Mighty AI, Scale and understand.ai are just a few that claim to have a more specialised labelling workforce. The differences between these crowd-powered endeavours and the mobilisation of crowds by CI projects are nuanced. It often comes down to the framing incentive structures, prioritisation between technology versus participant outcomes and their overall purpose (i.e. the aims they prioritise).
CI projects typically articulate a collective purpose, while paid crowd-labelling efforts emphasise individual gains by paying for each completed microtask. Research has shown that incentivising collective performance can lead to better outcomes by preserving the diversity of individual contributions; in contrast, individual incentives encourage increased conformity and impaired collective performance. On the other hand, some of the challenges faced by researchers working with crowds in either AI or CI show unsurprising convergence. For example, across both fields there is literature that addresses how to optimise participant performance and track the changing motivations of the crowd to maintain engagement.
How collective intelligence could help create better AI
There is no doubt that the costs and potential negative impacts of AI throughout its lifecycle deserve careful attention, but there is also a risk that these issues will overshadow the potential public benefits of the technology.
Collective intelligence offers an alternative path towards an AI-enabled future. By getting more of us involved in questioning AI, scrutinising its impacts and imagining which problems we should be applying it to, we can move towards more values-driven deployment of smart machines. Below, we outline how the principles and methods of CI can be used to counterbalance some of the limitations of AI and ultimately, improve its development.
Figure 5
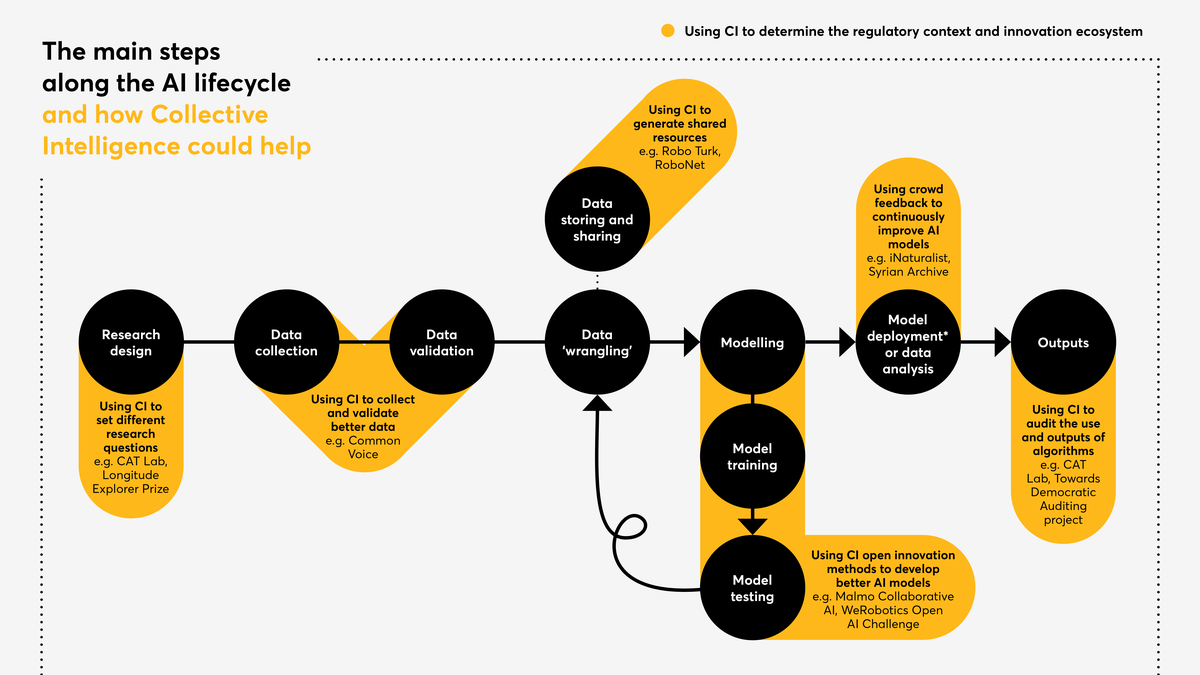
* Model deployment is the integration of a model into a real-world context for interaction with the target users. Common examples include search engines and recommender systems on entertainment platforms.
CI for better training data
Although crowdsourcing is already widely used within the AI community, it is rarely deployed with the explicit purpose of improving training data in order to develop technologies that have a wider public benefit and do not place under-represented groups at a disadvantage. One of the ways to develop fairer AI systems is to train them on datasets that are more representative of the diversity of real-world experience (an underlying principle of CI). This requires a more deliberate effort to involve individuals with rare knowledge, such as members of indigenous cultures or speakers of unusual dialects, in data collection. Mozilla’s Common Voice project uses an accessible online platform to crowdsource the world’s largest open dataset of diverse voice recordings, spanning different languages, demographic backgrounds and accents. Common Voice aims to open up the AI market and stimulate the development of AI voice assistants that are able to serve the needs of more diverse communities. Other projects focus on a smaller subset of languages; for example, Siminchikkunarayku and Masakhane focus on Peruvian and regional African languages respectively. These projects recognise that developing AI systems based on under-represented languages and voices helps to preserve and increase the reach of this unique cultural heritage. The resulting AI systems are also better able to serve the needs of these groups, who would otherwise be excluded.
All of these projects also integrate CI principles in their approach to data sharing by developing open, shared repositories of data. When data is rare, it is even more important to institute these practices so that more researchers and organisations can use the data to make progress. Sometimes the data required to train AI models is difficult to collect because it is very specialised. This is the case for autonomous systems in robotics, which rely on physical demonstrations by humans in order to develop machines that reproduce actions in the real (physical) world. Collecting demonstrations of tasks performed by people, whether recorded on video or through interactive displays, can be time consuming and cumbersome. The RoboNet open data repository, which brings together data collected by robotics labs from different institutions and the crowdsourcing platform RoboTurk, are two recent efforts by the robotics community to accelerate progress in the sector using CI.
Using CI to open up innovation
From peer knowledge production to targeted Challenge Prizes, many CI methods are concerned with harnessing the power of groups to generate new ideas or develop new solutions. The computer sciences and tech communities have embraced open innovation practices, such as the peer production of both code and models to accelerate the development of software. Dedicated open innovation platforms such as Kaggle and InnoCentive have made it simple to outsource difficult problems to distributed communities of experts, but it is rare for these communities to take explicit measures to ensure the diversity of their contributions. Some open-source software communities have even been accused of the opposite: promoting harmful power dynamics that discourage newcomers from contributing.
Some exceptions demonstrate how the principles of CI can be integrated into open innovation practices. The global peer learning community co‑ordinated by WeRobotics, regularly runs a Challenge Prize known as the Open AI Challenge to stimulate AI development around a specific issue that its members (the Flying Labs network) are facing. During the Tanzania Open AI Challenge in 2018 the organisation worked with local partners and non-profit groups to encourage participation from innovators based in or originating from Africa. Restricting competition entries to groups that are otherwise excluded from the innovation ecosystem can help broaden the range of ideas about how AI is developed and applied to solve societal problems. An example of this approach is Nesta’s Longitude Explorer Prize, which has been running since 2014 and targets applicants between 11 and 16 years old. It invites teams from schools in the UK to submit creative ideas for how to use AI to make progress on some of society’s biggest challenges, such as the climate crisis and ageing populations.
CI to audit and monitor AI
Some algorithmic methods are already being applied in complex social contexts that rely on value judgements and moral reasoning, such as: who should be granted early release from prison? Who has a right to social welfare? How do we prioritise housing allocation for those in need? Even if AI can help us assess and model these situations, we first need to have a wider conversation about the values we want technology to help promote and the level of responsibility that we think it is appropriate to delegate to autonomous systems as opposed to human judgement. To do this effectively, we need to use methods of collective sense-making that will allow us to interrogate algorithmic systems at all stages of the AI lifecycle – not just at the tail end, where algorithmic performance is judged by the outputs. To date, most public consultations about AI have focused solely on decision-making by AI systems or abstract notions of AI ethics rather than this more holistic practice-based approach (see Figure 5).
Light-touch auditing of AI performance already occurs in certain AI & CI project pipelines, for example if models are deployed for immediate use by communities in real-world contexts. By setting up a feedback loop from participants, models can continuously update their functions and iteratively improve to better serve the user’s needs. For example, iNaturalist, the social network used by nature lovers to learn about, discuss and share images of animals, has integrated an AI model that uses computer vision to help community members accurately classify each animal sighting they enter into the database. The classifications suggested by the model are more accurate for some species than others. When users come across an error in the algorithm’s classification, they can report it and assign the correct tag. This feedback loop ensures continuous collective oversight of the algorithm’s performance.
The next step is developing more sophisticated approaches to ‘collaborative governance’ of intelligent machines. CI could play a role throughout the AI pipeline, using active group deliberation to question assumptions and reach agreement about the use and performance of AI. This vision of collectively intelligent governance could take place on many levels, from legally binding multi-stakeholder public–private partnerships where organisations hold each other accountable, to the distributed moderation practices used by online communities. Importantly, these new models of CI-inspired governance to audit and monitor AI need to involve communities that are likely to be affected by the use of AI. CAT Lab uses citizen social science to examine the impacts of emerging technology (including AI) on online communities. In one research project, CAT Lab worked with an active community on Reddit[2] to learn about how communities can mitigate against the negative behaviours fuelled by the platform’s algorithms. The research, analysis and interpretation was carried out in collaboration with the communities. Towards Democratic Auditing is a project run by the Data Justice Lab in Cardiff that aims to develop processes for groups to monitor and take action on the use of automated scoring and decision-making systems by public sector organisations.
Auditing practices are also emerging among teams of domain experts in the context of automated professional DSTs. For example, social service workers responsible for child welfare risk assessments in Douglas County in the US have developed a new process (called the Red Team) where they undertake a team-based interpretation of algorithmic risk scores to guide their decision-making.
Diversity for better AI
Research on CI has shown time and again that a group’s diversity affects how well it is able to solve problems. This poses a significant challenge for AI, where diversity is notoriously lacking. In 2019, an analysis of the participation of women in AI found that of the 1.3 million articles published about AI only 13.8 per cent included a female author. The study also showed that women working in physics, education, computer ethics and other societal issues, and biology, were more likely to publish work on AI in comparison with those working in computer science or mathematics. Papers with at least one female co-author tended to be more focused on real world applications and used terms that highlighted the social dimensions of the research, such as fairness, human mobility, mental health and gender.
This illustrates an important point about widening participation in technology development and how a lack of diversity affects the kinds of questions that we apply AI to. The background and experiences of developers affect the way they frame problems or even how they code algorithms, as these may be based on the assumptions they hold. We stand to gain by taking active measures to embed diversity at all stages of the AI lifecycle, from the collection and validation of the data used to train algorithms all the way through to their interpretation and use. Although adding even more ‘messy’ real-world contributions may start to slow things down, it will help to make sure that the development of AI does not slide into a trajectory determined by a narrow demographic group. AI can only help to enhance CI if we make deliberate and thoughtful choices to ensure that it reflects the differences we see in society.
[1] This is known as supervised machine-learning.
[2] Reddit is an online discussion platform with over 13 million subscribers. The study described in the text worked with the r/science community.