We conclude with an exploration of the quality of AI research to tackle COVID-19 and the levels of experience and track record of AI researchers who are reorienting their activities towards COVID-19, also checking the extent to which doing so involves large ‘jumps’ from the area where they worked before.
Here, it is important to note that we are using citation data as an imperfect proxy for the quality and influence of a publication. While we try to account for differences in citation rates across scholarly communities, and over time, by comparing citation levels inside topical clusters and circumscribing our analysis to 2020, we recognise the limitations of this measure of research quality and impact – expanding this with other indicators is an important issue for further research.
In Figure 11 we compare the distribution of citations across article sources, between COVID-19 and non-COVID-19 articles, and between AI and non-AI papers. The reason to distinguish between article sources is that these are used by researchers in communities in disciplines with different citation approaches.
The chart shows that most publications in the data have not received any citations, which is perhaps to be expected given that we are only focusing on papers published this year. In general, papers in biomedical sciences (bioRxiv) and medical sciences (medRxiv) tend to receive more citations, specially if they focus on COVID-19: the share of medRxiv COVID-19 papers with at least one citation is over five times higher than the share of non-COVID-19 papers from that source. The share of biorXiv papers about COVID-19 with at least one citation is almost four times higher. By contrast, the proportions of papers with at least one citation in arXiv is similar between COVID-19 and non-COVID-19 papers. When we compare COVID-19 and non-COVID-19 AI papers from different sources, we find that those in bioRxiv and medRxiv tend to have a higher share of papers with at least one citation, while AI papers about COVID-19 from arXiv tend to receive 14 per cent less citations than those that are not about COVID-19.
These differences could be explained by variation in the interest and quality of AI papers to tackle COVID-19 generated by different communities. In particular, one might expect AI applications developed by biotechnologists and medical scientists to involve a higher level of subject expertise than contributions coming from computer science, enhancing the latter’s ability to tackle COVID-19. It could also be that AI research to tackle COVID-19 published by computer scientists has less visibility (for example, as we noted in the introduction, arXiv is not even included in the CORD-19 dataset of scholarly work to tackle COVID-19).
Figure 11: COVID-19 papers (both AI and non-AI) tend to receive more citations in the medical and biological sciences, but not in arXiv
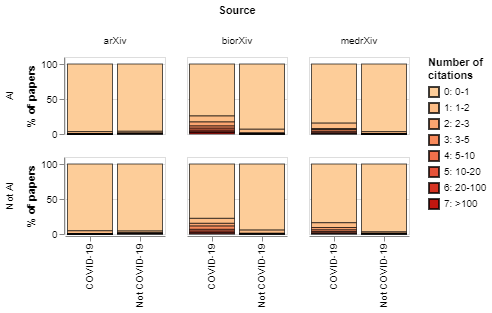
An interactive version of this graph is available in the online report
In figure 12 we compare the levels of citations across topical clusters and AI/non-AI categories. Each tick represents a paper, and the circles represent the number of citations received by papers at different levels. The charts are sorted in decreasing order of average citations. Clusters 12, 1 and 14, all of which relate to biomedical research, tend to receive the largest number of citations. Topical clusters dominated by AI such as cluster 16, cluster 19 and cluster 4 tend to receive less citations.
The citation distribution is highly skewed, with most papers receiving no citations at all and a handful of papers receiving hundreds of them. This makes it difficult to compare mean citation levels across groups. Having said this, we find that in only three of the top 12 topical clusters by levels of AI activity, the average citation counts for AI papers are higher than for non-AI papers (they are cluster 18, involving predictive analyses of hospital data, cluster 12, about vaccine discovery, and cluster 25, about COVID-19 diagnosis).
Figure 12: Number and frequency of citations for clusters with high levels of AI activity
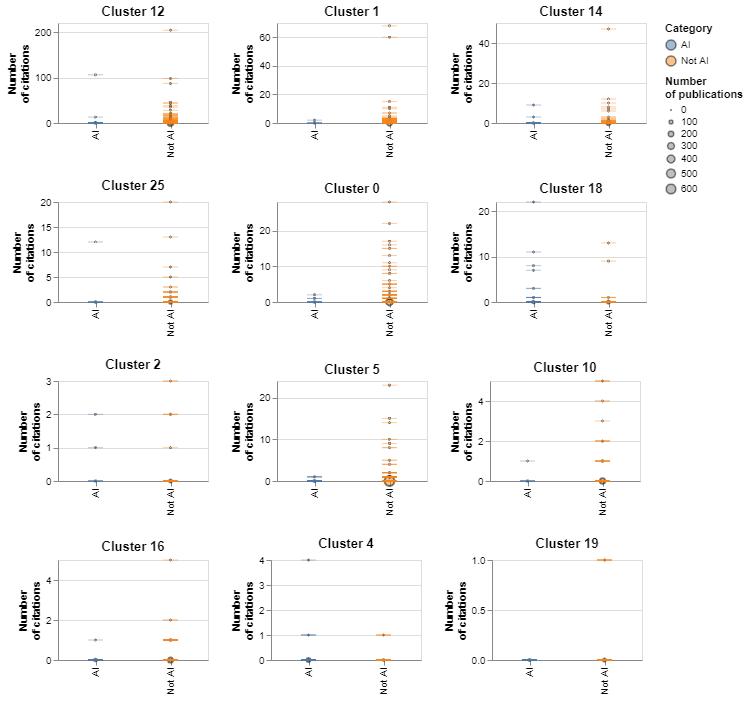
An interactive version of this graph is available in the online report
We have also studied the experience and track record of researchers participating in COVID-19 research. In order to do this, we look at the number of papers, and mean and median number of citations received by papers involving an author before 2020, focusing on recent publications (i.e. publications in 2018 or 2019). We then compare the means for those statistics between different groups of researchers, also testing whether any differences are statistically significant.[4]
In Figure 13 we compare our indicators of track record between researchers that have recently focused their work on COVID-19 and those that have not (first row), AI researchers that have focused on COVID-19 and those that have not (second row) and researchers tackling COVID-19 with or without AI (third row). We colour the bars based on the statistical significance of the results. The figure shows that:
- In general, researchers tackling COVID-19 have stronger track records: they have published more papers in recent years and those papers tend to receive more citations.
- When we focus on AI researchers, we find that researchers tackling COVID-19 tend to be more prolific and somewhat less likely to be cited (although some of the differences are not statistically significant).
- When we compare researchers tackling COVID-19 with AI with those using other techniques, we find that although AI researchers tend to be more prolific, they have a weaker track record, tending to have received less citations in previous work.
Figure 13: AI researchers tackling COVID-19 tend to have a less established track record (in terms of past citations) than non-AI researchers
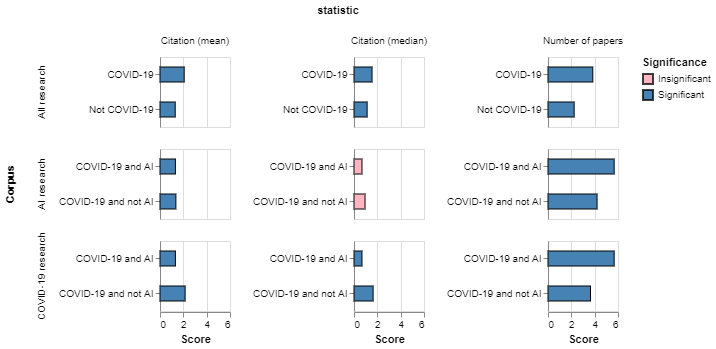
An interactive version of this graph is available in the online report
As before, there is the risk that some of the differences highlighted above may be driven by variation in citation propensities across research communities and disciplines, or varying levels of interest in research across domains. To account for this, in Figure 14 we compare the recent track record of AI and non-AI researchers active in the same topical cluster. We use the opacity of the bars to convey whether the differences being displayed are statistically significant or not.
We find that although researchers using AI to tackle COVID-19 tend to be somewhat more prolific (their mean number of publications is higher for 60 per cent of the clusters we consider), they are less established in their track records (in 90 per cent of the clusters non-AI researchers have higher citation medians, and in 80 per cent they have higher citation averages). All the statistically significant differences in citations are in favour of non-AI researchers, suggesting that they have authored more influential research in recent years.
Figure 14: AI researchers tend to have less established records when we compare them with non AI-researchers in the same topical cluster
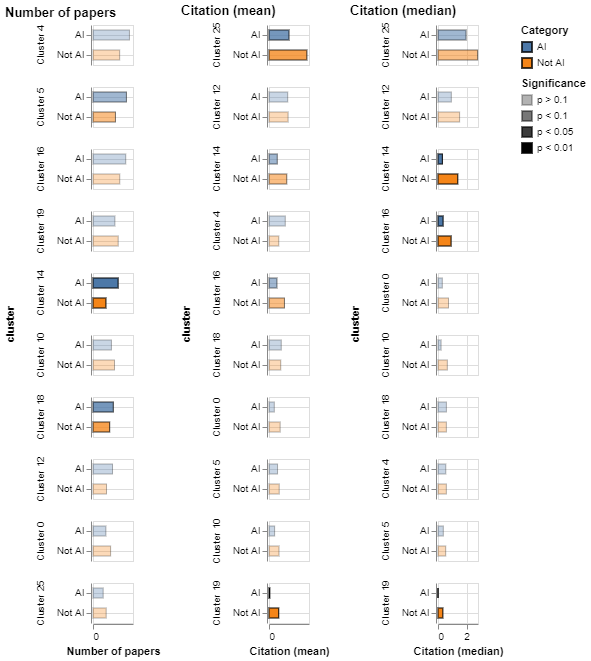
An interactive version of this graph is available in the online report
Lastly, we analyse the content of the contributions of AI researchers to examine their thematic diversity and how it changed due to publishing COVID-19-related work. In Figure 15 we show that COVID-19 publications increased the thematic diversity of most AI researchers. This increase is higher for those with less than 10 citations in total. We also find that COVID-19 publications increased the thematic diversity of AI researchers working in health while we identify a group of interdisciplinary researchers whose thematic diversity was already high and COVID-19 work did not alter it significantly. These findings are consistent with our citation analysis; researchers who were specialised on core AI and computer science topics made longer ‘jumps’ to contribute to COVID-19 than those with a cross-disciplinary profile.
Figure 15: Length of thematic jumps for AI researchers becoming active in COVID-19 research
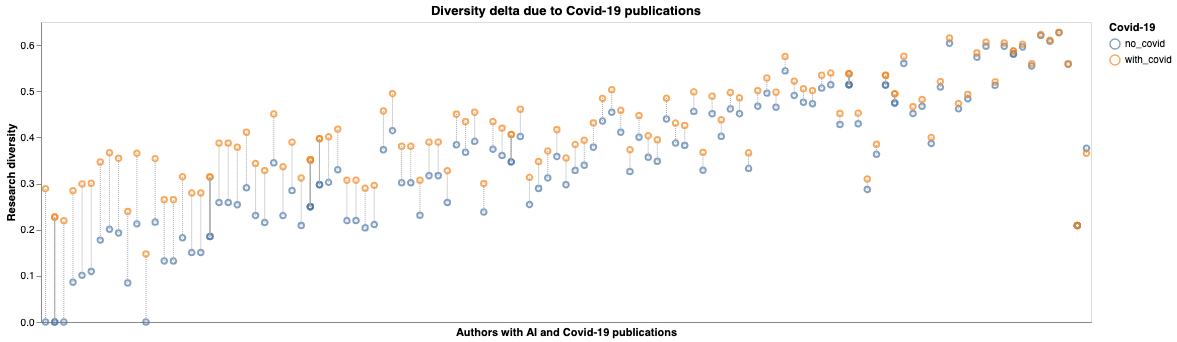
Footnotes
4. We do this using the Mann-Whitney test, which does not assume normality in the distribution of the statistics we are comparing.