Executive summary
ArtificiaI intelligence (AI) techniques could play an important role in the mission to tackle COVID-19, from helping to discover new drugs and vaccines to predicting the spread of infection and testing patients. At the same time, many AI techniques are experimental, rely on big, sensitive datasets and might be difficult to apply in high-stakes domains such as health.
This report studies the levels, evolution, geography, knowledge base and quality of AI research in the COVID-19 mission field using a novel dataset taken from open preprints sites arXiv, bioRxiv and medRxiv, which we have enriched with geographical, topical and citation data.
Findings
Although there has been rapid growth in the levels of AI research to tackle COVID-19 since the beginning of the year, AI remains underrepresented in this area compared to its presence in research outside of COVID-19. So far in 2020, 7.1 per cent of research on COVID-19 references AI, while 12 per cent of research on topics outside COVID-19 references it. After growing rapidly earlier in the year, the share of AI papers in COVID-19 research has stagnated in recent weeks.
More than a third of publications to tackle COVID-19 involve predictive analyses of patient data and in particular medical scans. AI is also being deployed to analyse social media data, predict the spread of the disease and develop biomedical applications.
China, the US, the UK, India and Canada are the global leaders in the development of AI applications to tackle COVID-19 research, accounting for 62 per cent of the institutional participations for which we have geographical data. China in particular is overrepresented in COVID-19 AI research. We have also identified a substantial number of publications involving institutions that we are unable to match with the global research institution database we are using. This is consistent with the idea that new actors are entering the COVID-19 mission field.
AI and non-AI researchers working in COVID-19 tend to draw on different bodies of knowledge. AI’s share of citations to computer science is five times higher than outside and its share of citations to medicine is a third lower. These differences hold, even after we control for the topic within COVID-19 that different publications focus on .
In general, AI papers to tackle COVID-19 tend to receive less citations than other papers in the same topic. The population of AI researchers active in the COVID-19 mission field also tends to have a less established track record proxied through the citations they have received in recent years. This result holds when we compare researchers working in the same topics, suggesting that it is not simply driven by variation in the citation behaviours of different communities and disciplines.
Implications
Our analysis highlights the velocity with which research communities – including AI researchers – are mobilising to tackle the COVID-19 pandemic. We find many opportunities to apply powerful AI algorithms to prevent, diagnose and treat the virus. At the same time, deep learning algorithms’ reliance on big datasets, difficulties interpreting their findings, and a disconnect between AI researchers and relevant bodies of knowledge in the medical and biological sciences may limit the impact of AI in the fight with COVID-19. The persistent underrepresentation of AI research in the COVID-19 field we evidence, and its focus on computer vision analyses that play to the strengths of current algorithms, but require substantial investments in hardware and changing how hospitals work, are consistent with the notion that AI’s may play a limited role tackling this pandemic.
There is also the risk that researchers facing low barriers to entry into the field may produce low-quality contributions making it harder to find valuable studies and discourage interdisciplinary contributions that could take longer to develop. Our finding that AI research tends to be less cited than other research, even inside the same publication topics, and that AI researchers entering the field have a weaker track record on average than others, lends some support to these concerns.
How can we harness AI’s potential to tackle COVID-19 and future pandemics, while removing some of its risks?
In the shorter term, creating bigger higher-quality open datasets related to COVID-19 could make it easier to deploy state-of-the-art deep learning algorithms. Spurring interdisciplinary collaborations, bringing together AI researchers and subject experts, may help to prioritise those AI applications with the greatest relevance and value. It might also reduce the risk of ‘AI imperialism’; where AI researchers ignore relevant bodies of knowledge about the complex biological and social systems where their techniques will be applied, reducing their value and creating unintended consequences. We also need technological and social solutions for the challenge of navigating a vast and fast-growing body of research of uncertain quality. Going forward, research funders should encourage the development of AI algorithms that are easier to deploy in small-data, high-stakes domains.
Novel data sources and methods, such as those we have used in our analysis, can play an important role in informing these strategies.
The data set used in this report is open for other researchers to analyse and build on.
Introduction
Artificial Intelligence (AI) is the discipline that seeks to develop technologies that are able to adapt their behaviour in response to different situations, thus imitating the flexibility and general-purpose nature of human intelligence (Markoff, 2016; Mitchell, 2019; Wooldridge, 2020).
In recent years, the field has adopted a machine learning paradigm where the AI systems it develops ‘learn’ patterns from examples (generally big datasets labelled with features of interest) or by exploring simulated environments (Russell & Norvig, 2016; Sutton & Barto, 2018). This is underpinned by deep learning algorithms able to extract structure from complex data such as images, video, sound, language and networks to make accurate predictions (Goodfellow et al., 2016).
These techniques have contributed to important advances in computer vision, natural language processing, robotics and scientific research and development (R&D), leading economists to recognise AI as a general purpose technology with transformational potential (Cockburn et al., 2018; Klinger et al., 2018). This has been accompanied by growing levels of funding, investment and public, and policy interest in these powerful technologies.
AI systems are also expected to make important contributions across the health landscape, improving drug discovery, disease prevention, diagnosis and treatment and operational efficiency (Mateos-Garcia, 2019; Ravì et al., 2016; E. Topol, 2019; E. J. Topol, 2019). In recent months, researchers have identified several opportunities to apply AI or machine learning to tackle the COVID-19 pandemic, with a recent review showcasing examples of these applications in the molecular, clinical and social domains (Bullock et al., 2020; Naudé, 2020a; van der Schaar & Alaa, 2020).
In this paper, we build on these reviews to quantitatively map the levels of AI research activity to tackle COVID-19 using data from three widely used preprints repositories – arXiv, bioRxiv and medRxiv. Critically, in addition to measuring the volume of AI research to tackle COVID-19 and its evolution in recent months, we also use various data science techniques to decompose the field into application areas where AI systems are being developed or deployed to tackle COVID-19. We also analyse the geography of AI research and the knowledge base on which it builds, and study the trajectory of the research teams working in the field.
This way, we aim to get a more granular understanding of the AI response to COVID-19 and some of its potential limitations. This is informed by the idea that, despite their generality, modern AI techniques underperform in the absence of big datasets or where data exists but cannot be used for analysis, for example for privacy reasons, and that the predictions they generate are often difficult to explain and interpret, rendering them less useful for high-stakes health decisions where accountability is key (Marcus, 2018). Researchers and journalists who are assessing potential pitfalls in the AI response to COVID-19 have already pointed at these issues (Bullock et al., 2020; Naudé, 2020b). Through our analysis of the composition of research in this area, and how it compares to broader research related to COVID-19, we aim to evidence the situation quantitatively.
Our analysis also connects with an emerging body of work about the response of the research and innovation (R&I) system to COVID-19. This work highlights the velocity with which research communities from many different disciplines have reoriented their efforts in a collective mission to tackle COVID-19. Their strong problem-focus may encourage interdisciplinary collaboration, help deploy technologies such as AI in the health sector and bring new actors into the R&I system (Younes et al., 2020).
At the same time, some scholars have raised concerns about the consequences of this COVID-19 ‘research rush’. Research during this period may have lower standards of review, which could result in low-quality and even risky research being published; a focus on short-term research agendas by fast-moving players, which may discourage entry by other organisations with longer-term goals and more rigorous methods and cultures (Bryan et al., 2020); and contributions to the debate that are not sufficiently informed from researchers without expertise in relevant fields such as epidemiology. We seek to evidence these issues through our analyses of the quality of AI research to tackle COVID-19 (which we proxy through citations) and the track record of participants in the field.
By looking at the knowledge base of AI research to tackle COVID-19, we also attempt to measure the extent to which AI researchers are incorporating knowledge from other disciplines in their work or not. In doing this, we build on previous studies suggesting that AI research tends to be disengaged with other research fields (Frank et al., 2019).
The code and data underpinning the paper can be accessed from this GitHub repository.
Data sources and methodology
Data sources
A full list of Data sources, and their strengths and weaknesses, are listed below.
Our core dataset comprises 1.8 million papers from arXiv, bioRxiv and medRxiv, three preprints repositories respectively used by researchers in science, technology, engineering and maths (STEM) subjects (including computer science), biological sciences and medical sciences to disseminate their work prior to publication. There are several reasons why we have opted for this data instead of alternative data sources such as the CORD-19 dataset about COVID-19 related research, released by Semantic Scholar in partnership with leading data providers.
- Researchers increasingly use preprints sites to share their results close to real-time, speeding up the dissemination of findings that may be relevant to tackle COVID-19. (Younes et al., 2020). At the same time, lower thresholds to publication might create quality problems such as those we mentioned in the introduction. This makes these datasets a good setting to explore the positives and negatives of the COVID-19 research rush that we have witnessed in recent months.
- The CORD-19 dataset does not include any data from arXiv, yet as we demonstrated in previous work, this is an important outlet for the dissemination of artificial intelligence (AI) research by leading teams and private research labs (Klinger et al., 2018). We believe that incorporating it into the analysis will improve our coverage of AI research to tackle COVID-19.
- Working with the whole corpus of AI research in arXiv, bioRxiv and medRxiv allows us to compare the topical, geographical and institutional composition of AI research to tackle COVID-19, with the broader AI research domain (as captured by those sources) helping us measure what parts of AI research and what countries are over or underrepresented in the fight against COVID-19.
Having collected the core data, we have fuzzy-matched it on paper titles with Microsoft Academic Graph (MAG), a bibliometric database hosted by Microsoft Academic that currently contains 236 million publications (Wang et al., 2020).[1] This allows us to extract the institutional affiliations of a publication’s authors, the fields of study for publications (which Microsoft extracts from their text using natural language processing) and their citations to other papers for a subset of them (we consider the representativeness of this sample in Findings – Knowledge creation and combination).
We use the Global Research Identifier Database (GRID), an open database with metadata about around 97,000 thousand research institutions to further enrich the dataset with geographical information, specifically a latitude and longitude coordinate for each affiliation that we can then geocode into countries and regions. The GRID data is particularly useful since it provides institute names and aliases (for example, the institute name in foreign languages).
Each institute name from MAG is matched to the comprehensive list from GRID (Klinger et al., 2018). In a number of instances, our approach fails to produce a valid match either because the original paper did not include information about an institute, because of inconsistencies in the formatting of institute names, or because the institutes that participated in a paper are not present in the GRID database. A higher matching rate in a field suggests that it involves traditional research institutions more likely to be captured by GRID, and lower matching rates points at the participation of new entrants and institutions.
We conclude our summary of the data by noting that the top 10 institutions publishing COVID-19 related papers in our dataset are Harvard University, Huazhong University of Science and Technology (based in Wuhan, ‘ground zero’ for the pandemic), University of Oxford, Wuhan University, Fudan University, the Chinese Academy of Sciences, Stanford University, the National Institutes of Health, Centers for Disease Control and Prevention and Imperial College London. This supports the idea that our data includes research outputs from highly reputable and relevant research institutions we would expect to be making important and timely contributions to tackling COVID-19.
Data set: arXiv articles
Source: arXiv
Strengths: A single established repository of preprints for quantitative subjects, which is almost guaranteed to contain seminal works in fields such as AI.
Weaknesses: Data set is not intrinsically linked or enriched, so one must obtain geography, citation information and fields of study, or other enrichments, using other sources.
Data set: bioRxiv/medRxiv articles
Source: MAG
Strengths: Open repository of preprints which has seen exponential growth during the COVID-19 pandemic.
Weaknesses: Relatively small number of articles and the coverage of high-quality research is not known.
Data set: Geography
Source: GRID
Strengths: Institute matching for typical analyses is around 90 per cent.
Weaknesses: Anecdotal evidence that coverage loss is biased against institutions in developing nations.
Data set: Citations
Source: MAG
Strengths: Has a large user base, so their procedure is likely to be ‘battle hardened’ in terms of iterations.
Weaknesses: Citations are not normalised, to account for the field of study for example. Anecdotal evidence that coverage loss is biased against institutions in developing nations.
Data set: Fields of study
Source: MAG
Strengths: Extensive coverage of disciplines.
Weaknesses: Based on a hierarchical topic model. It is unclear what the caveats in their methodology are. Citations are not normalised, to account for the field of study for example. Anecdotal evidence that coverage loss is biased against institutions in developing nations.
Methodology used to identify COVID-19 and AI
We identify COVID-19 publications by following the same approach used by arXiv. We search for the terms ('SARS-CoV-2', 'COVID-19', 'coronavirus') in either the main body or title of an article. If any of them is found, we assume that the paper is related to COVID-19. This approach leads to identifying 5,450 COVID-19 related papers in our data.
We identify AI publications in bioRxiv, medRxiv and arXiv using a keyword-based approach. We process 1,789,542 documents and find short phrases in them (bigrams and trigrams). Then, we train a word2vec model, a method that finds a numerical representation of words based on their context. We query the trained word2vec with a list of AI terms such as ‘artificial intelligence’ and ‘machine learning’, and pick the most similar words to them. This query-expansion approach produces a list of terms (see annex) that we search for in the preprocessed documents. If any of them is found, we label the document as AI. This way, we identify 82,434 AI papers in the data.
Other analytical techniques used
Topic modelling
In broad terms, topic modelling algorithms exploit word co-occurrences in documents to infer distributions of words over topics (conceived as clusters of words that capture a theme in a corpus) and distributions of topics over documents (capturing the range of themes covered in each document).
Here, we analyse publication abstracts with Hierarchical Top-SBM. This algorithm, based on the stochastic block-model used in network analysis, transforms a corpus into a bipartite graph where words are connected based on their co-occurrence in documents, and documents are connected based on the words that co-occur in them (Gerlach et al., 2018). These two graphs are decomposed using community detection to extract communities of words (topics) and communities of documents (clusters). We use this approach to classify documents into topical clusters that are informative about the focus of a publication based on its abstract, and interpret the clusters based on those topics that are salient in them (we describe the findings in Findings – Topical composition).
Two additional advantages of Top-SBM over other topic-modelling algorithms such as Latent Dirichlet allocation is that it detects the number of topics (and conversely clusters) in a document automatically and makes more realistic assumptions about the distribution that generates the corpus.
Analysis of distance
In Findings – Quality, we explore the impact of publishing COVID-19 on the thematic trajectory of researchers. We frame this inquiry in terms of research diversity: assuming that researchers publish mainly in one broad field (for example, computer vision), publishing a paper in epidemiology and COVID-19 would increase their research diversity as it would be thematically different from the rest of their outputs. We operationalise this idea by focusing on author-level contributions.
We keep the subset of authors that had at least two AI papers and one related to COVID-19 in order to measure the research diversity of their AI publications and how it changed after publishing COVID-19 work. The larger the change, the further out of their previous thematic focus the COVID-19 publications are.
We quantify author-level thematic diversity as follows:
diversity = iN(cosine_distance(vi, centroid))N
Where vi is the vector of paper i, N is the total number of an author's papers and the centroid is the average vector of papers for that author.
- See Klinger et al., (2018) for a summary of the algorithm we use for this matching.
Findings – Levels and evolution of activity
Our analysis of just under 1.8 million papers with abstract information in arXiv, bioRxiv and medRxiv reveals 82,423 artificial intelligence (AI) papers (4.6 per cent of the total), 5,450 COVID-19 papers (0.3 per cent of the total) and 379 AI papers that focus on COVID-19 (0.21 per cent of the total).
In order to study the representation of AI in COVID-19 research, we subset the data to focus on 2020 when 98.7 per cent of the COVID-19 papers in our data were published and compare the share of AI papers in non-COVID-19 research with their share outside COVID-19 research.
As Figure 1 shows, research relating to COVID-19 is less likely to deploy AI techniques than research outside this topic. Just 7.12 per cent of papers about COVID-19 mention AI, whilst for papers that are not about the pandemic the figure increases to 12.49 per cent. The presence of AI research in COVID-19 is 43 per cent lower than we would expect if AI was present there at the same frequency as in other topics.
Figure 1: The share of AI research inside COVID-19 is lower than the share outside

An interactive version of this graph is available in the online report
When we consider various trends of interest in Figure 2, we find that the share of COVID-19 activity in our corpus has been growing rapidly to reach around 10 per cent of all research in our corpus, highlighting the velocity with which the scientific community has reoriented its efforts to tackle COVID-19, and the importance of preprints as an outlet to disseminate their efforts. COVID-oriented AI research has also grown since around March 2020, although it has stabilised now at around 6 per cent of all of all AI research. Interestingly, the share of AI research in papers about COVID-19 research is stagnant or even declining after a peak of activity towards the end of March 2020.
Figure 2: COVID-19 research is gaining importance but the share of AI in it is stable or even stagnant
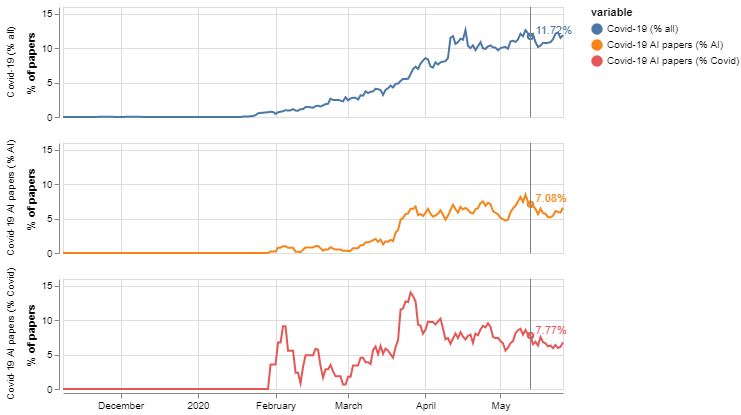
Figure 2: COVID-19 research is gaining importance but the share of AI in it is stable or even stagnant
We also consider the share of papers in categories of interest accounted for by different article sources (arXiv, bioRxiv and medRxiv). As Figure 3 shows, arXiv accounts for more than 90 per cent of AI papers in our corpus but only 20 per cent of the COVID-19 papers. When we look at the intersection between both categories, we find that arXiv accounts for half of the AI papers to tackle COVID-19, and medrXiv and biorXiv for the rest. This is consistent with the idea that researchers from various disciplines are exploring ways to deploy AI research in order to tackle COVID-19, and justifies our decision to incorporate arXiv into the analysis.
Figure 3: Share of relevant categories accounted by different sources
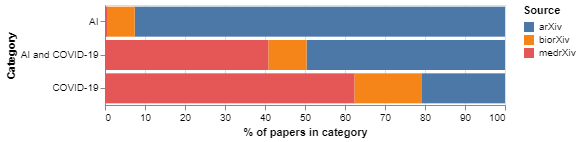
An interactive version of this graph is available in the online report
Findings – Topical composition
We use topSBM, a topic modelling algorithm, to analyse the composition of COVID-19 research and identify topical clusters based on the language in their abstracts. This analysis reveals 31 topical clusters involving 193 topics.[2]
The bar chart at the top of Figure 4 shows the share of all artificial intelligence (AI) and non-AI COVID-19 papers in different clusters, and the heat map below shows how prevalent are different topics (which we summarise with their most salient terms) in those topical clusters . For example, the heatmap shows that a topic that uses the terms ‘classification, images and deep learning’ is prevalent in Cluster 4, and a topic related to ‘Social Media, Twitter and tweets' is prevalent in cluster 16.
Highly-cited examples of AI research in different topical clusters summarises the content of some of the topical clusters with the highest levels of AI activity and gives examples of highly-cited AI publications in those clusters.
The first thing to note is some overlap in the themes of clusters, consistent with the idea that it is not trivial to classify many publications into a single area of activity. Having said this, we note that AI applications in the COVID-19 mission field include:
- Predictive analyses of medical scans to diagnose COVID-19 (clusters 4, 18 and 19).
- Analyses of digital (e.g. social media) data and development of digital solutions such as apps (cluster 16).
- Predictive analyses of the spread of the pandemic (clusters 5 and 10).
- Biomedical research for drug and vaccine discovery (clusters 12 and 14).
Over a third of AI applications to tackle Covid involve predictive analyses of patient data.
In relative terms, AI is overrepresented among predictive analyses of image data (where almost all publications involve AI), as well as analyses of social media data. Although there is a large number of AI papers analysing the spread of COVID-19 (cluster 5), they are in the minority in that category.
Figure 4: AI research to tackle COVID-19 focuses on predictive analyses of patient (specially imaging) data and analyses of social media data
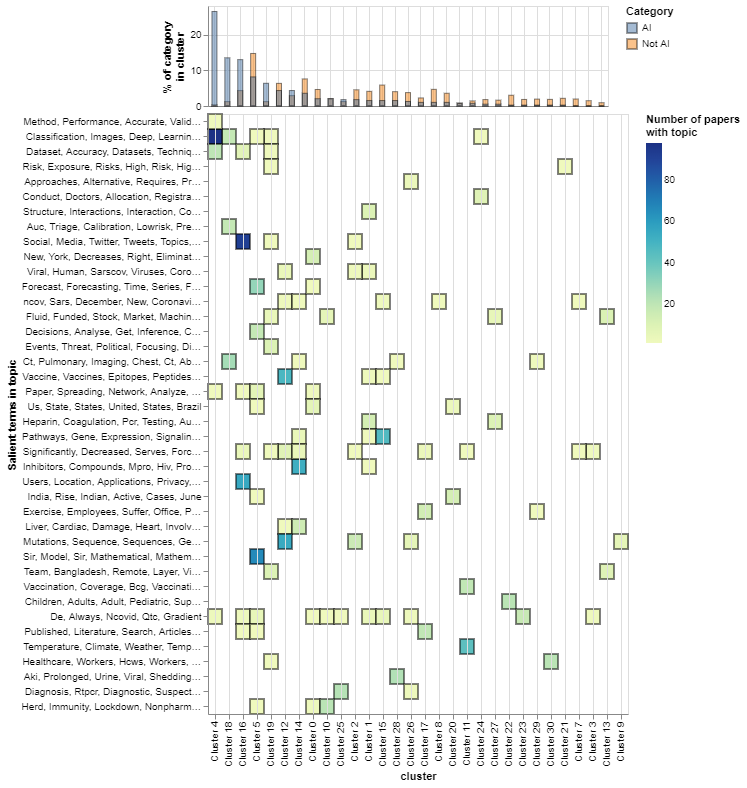
An interactive version of this graph is available in the online report
Cluster 4
Example AI papers:
AI-assisted CT imaging analysis for COVID-19 screening: Building and deploying a medical AI system in four weeks
COVID-CT-Dataset: A CT Scan Dataset about COVID-19
Automated Methods for Detection and Classification Pneumonia based on X-Ray Images Using Deep Learning.
Cluster 18
Example AI papers:
A deep learning algorithm using CT images to screen for Corona Virus Disease (COVID-19)
Deep learning-based model for detecting 2019 novel coronavirus pneumonia on high-resolution computed tomography: a prospective study
Deep learning-based Detection for COVID-19 from Chest CT using Weak Label
Cluster 16
COVID-19 Public Sentiment Insights and Machine Learning for Tweets Classification
A Machine Learning Application for Raising WASH Awareness in the Times of COVID-19 Pandemic
A Novel AI-enabled Framework to Diagnose Coronavirus COVID 19 using
Smartphone Embedded Sensors: Design Study
Cluster 5
COVID-19 Outbreak Prediction with Machine Learning
COVID-19 Infection Forecasting based on Deep Learning in Iran
Generating Similarity Map for COVID-19 Transmission Dynamics with
Topological Autoencoder
Cluster 19
Deep Learning System to Screen Coronavirus Disease 2019 Pneumonia
EXPLAINABLE-BY-DESIGN APPROACH FOR COVID-19 CLASSIFICATION VIA CT-SCAN
A Machine Learning Solution Framework for Combating COVID-19 in Smart Cities from Multiple Dimensions
Cluster 12
Severe acute respiratory syndrome-related coronavirus - The species and its viruses, a statement of the Coronavirus Study Group
Host and infectivity prediction of Wuhan 2019 novel coronavirus using deep learning algorithm
COVID-19 coronavirus vaccine design using reverse vaccinology and machine learning
Cluster 14
Predicting commercially available antiviral drugs that may act on the novel coronavirus (2019-nCoV), Wuhan, China through a drug-target interaction deep learning model
Potentially highly potent drugs for 2019-nCoV
Large-scale ligand-based virtual screening for SARS-CoV-2 inhibitors using deep neural networks
Cluster 0
Impacts of Social and Economic Factors on the Transmission of Coronavirus Disease 2019 (COVID-19) in China
Finding an Accurate Early Forecasting Model from Small Dataset: A Case of 2019-nCoV Novel Coronavirus Outbreak
Quantifying the effect of quarantine control in Covid-19 infectious spread using machine learning
Cluster 10
COVID-19 Epidemic in Switzerland: Growth Prediction and Containment Strategy Using Artificial Intelligence and Big Data
Coronavirus Geographic Dissemination at Chicago and its Potential
Proximity to Public Commuter Rail
COVID-19 and Company Knowledge Graphs: Assessing Golden Powers and
Economic Impact of Selective Lockdown via AI Reasoning
Figure 5 presents the sources for articles in different clusters, distinguishing between AI and non-AI publications in each row. Interestingly, it shows that although clusters 4 and 18 focus on a similar topic (predictive analyses of image data), the former is dominated by publications from arXiv (which we assume primarily involve computer scientists) while the latter is dominated by medRxiv (which we assume must involve medical scientists). Cluster 16, about analyses of social media data, is also dominated by publications from arXiv, while clusters 5 and 19 include a mix of computer science and medical science publications. AI papers focused on drug discovery (cluster 12) generally come from biorXiv. Interestingly, cluster 14, also about biomedical applications, includes many contributions from arXiv.
Figure 5: What disciplines are focusing on what topical clusters of AI research?
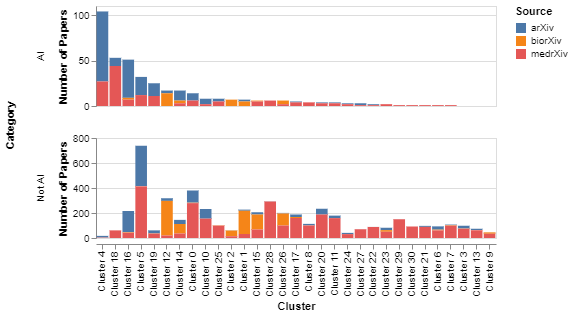
An interactive version of this graph is available in the online report
Footnotes
2. We have removed 38 very generic topics that tend to appear in most papers.
Findings – Geography
We have matched the institutional affiliations of the papers in our corpus with the Global Research Identifier (GRID) in order to analyse the geography of artificial intelligence (AI) research to tackle COVID-19.
The left point chart of Figure 6 shows the share of all activity in our corpus, AI research in general and AI research to tackle COVID-19 accounted for by the top 25 countries by size in the corpus.
First, we note that in a significant number of cases we are not able to match the institution involved in a paper with GRID – we label these cases as ‘unmatched’. Interestingly, matching rates are much higher for COVID-19 research in general than for AI research and COVID-19 oriented AI research, where we are unable to match institutions with GRID in almost 20 per cent of the cases. One interpretation for this is that COVID-19 research, in general, involves more and better known academic institutions likely to be captured by GRID, while the latter involves other actors, potentially new entrants in the research ecosystem who perhaps benefit from lower barriers to entry due to widespread availability of data and algorithms. Understanding who these institutions are and the factors driving their participation in research to tackle COVID-19 is an important question for future research.
Figure 6: The US and China dominate AI applications to tackle COVID-19, and we identify many new (unmatched) actors active in the field
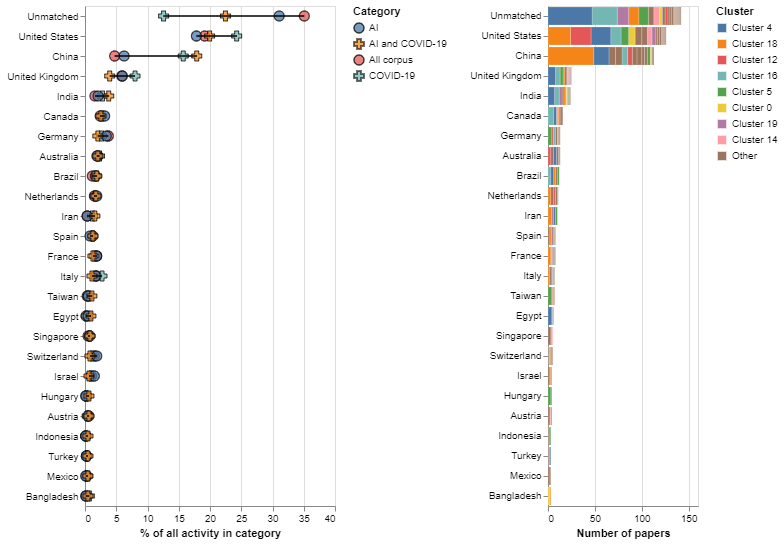
An interactive version of this graph is available in the online report
Focusing on those institutions that we have been able to match with GRID, we find that the five most active countries in the deployment of AI to tackle COVID-19 are the US and China, which together account for more than 40 per cent of research in this area.[3] They are followed by the UK, India, Canada and Germany – all countries that rank highly in most AI research maps (Klinger et al., 2018).
China’s share of COVID-19 and COVID-19 oriented AI activity is higher than we would expect, given its overall presence in the data, which suggests a strong focus on these subjects in that country.
Leaving out unmatched institutions, we find that 62 per cent of all AI research to tackle COVID-19 concentrates in the top five countries, which is similar to the concentration of COVID-19 research, and 12 per cent higher than the share of all activity in our corpus accounted for by the top five countries.
The right bar in Figure 6 presents the number of research participations in papers in different topical clusters by country, also including unmatched institutions. We see clear international differences in the topical focus of AI research to tackle COVID-19. For example:
- Unmatched institutions primarily focus on computer vision with medical scans (cluster 14) and analyses of social media data (cluster 16) – these are AI applications with relatively low ‘barriers to entry’.
- The United States and China are more focused on the topical cluster of computer vision that involves medical scientists (cluster 18).
- We also detect higher levels of AI biomedical activity (cluster 12) and analyses of the transmission of COVID-19 (cluster 0) in the US.
Figure 7 presents the evolution of participations in COVID-19 research from institutions in different countries. We see some evidence that COVID-19 related research tracks awareness of the disease in a country: China was the first country to reach more than 25 per cent of its COVID-19 related research activity (in early March 2020), followed by Iran three weeks later, and Canada and the US a month later. European countries and the UK have been somewhat slower in reaching that threshold, if only by a few days.
Figure 7: Evolution of activity in different countries
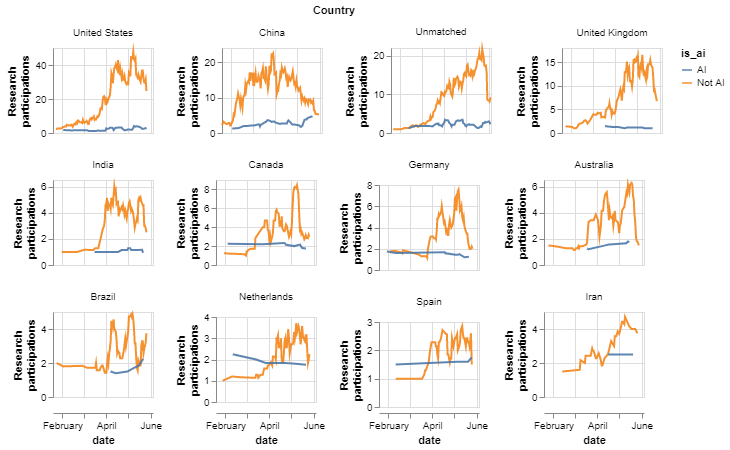
An interactive version of this graph is available in the online report
Footnotes
3. By participation, we mean participation of an institution from a country in a paper.
Findings – Knowledge creation and combination
Here, we focus on the knowledge base for artificial intelligence (AI) research to tackle COVID-19. We are particularly interested in determining the extent to which AI draws on subject expertise from medicine and the biological sciences as well as computer science disciplines. In order to explore this question, we have extracted information about 22,404 papers cited by around 3,000 papers in our COVID-19 corpus.
We begin by comparing the share of citations to papers in various high-level disciplines from AI and non-AI research. Figure 8 presents the share of all citations from AI and non-AI COVID-19 papers accounted for by papers in various fields of study at the highest level of aggregation in Microsoft Academic Graph’s taxonomy.
It shows that computer science papers account for almost half of the citations from AI papers (five times higher than the share outside of AI). By contrast, the share of citations to medicine from AI papers is 35 per cent lower than in non-AI papers and the share of citations to biology papers is 65 per cent lower. This suggests that AI COVID-19 research draws on a different body of knowledge from non-AI research. Further confirming this idea, we find that AI and non-AI research to tackle COVID-19 only share 3.7 per cent of their references.
Figure 8: AI research tends to draw strongly on computer science, and much less on other disciplines such as medicine and biological sciences
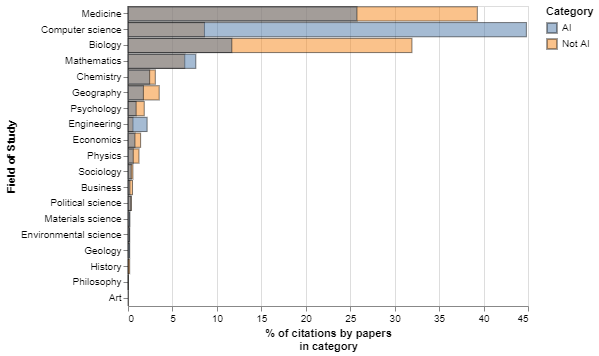
An interactive version of this graph is available in the online report
The result we reported above could reflect specialisation of AI and non-AI research in different topics drawing on distinct bodies of knowledge. To account for this, we compare the citation propensities of AI and not AI papers in the same topic, drilling down one level further from disciplines into subfields of research. We show the results in Figure 9.
There, we compare the share of citations to different sub-fields of study by AI and non-AI papers in different topical clusters. We focus on topical clusters with high levels of AI activity and on the 30 most highly cited fields of study in the COVID-19 corpus of research We colour the points in the chart to represent the higher-level field of study to which each subfield belongs.
Figure 9: AI and non-AI papers cite different subfields of research even when they are in the same topic
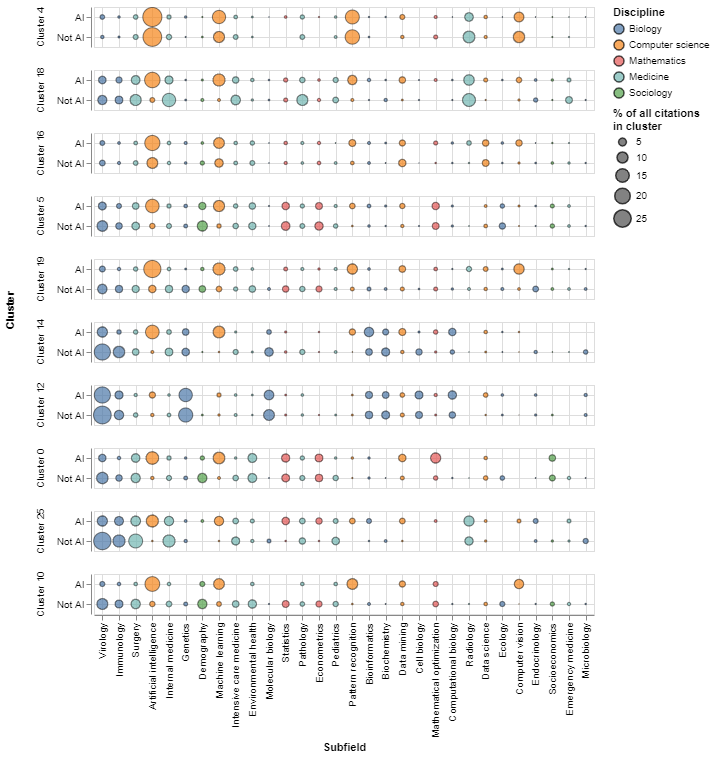
An interactive version of this graph is available in the online report
The figure shows important differences in cited subfields between AI and non-AI research, even inside the same topical cluster. For example, non-AI papers in cluster 19 focus their citations much more strongly on internal medicine, intensive care medicine and pathology while AI focuses on machine learning, pattern recognition and computer vision. In cluster 14 (about drug discovery), we see that a larger share of non-AI citations go to immunology, surgery and internal medicine, and molecular biology.
Consistent with this, and as Figure 10 shows, we find that the levels of overlap between papers cited in AI and non-AI research in the same topical cluster tend to be low, ranging between 5-8 per cent for computer vision papers and zero for several clusters (admittedly, some of these do not include any AI papers).
Figure 10: There are very low levels of overlap between the AI and non-AI references in different topical clusters
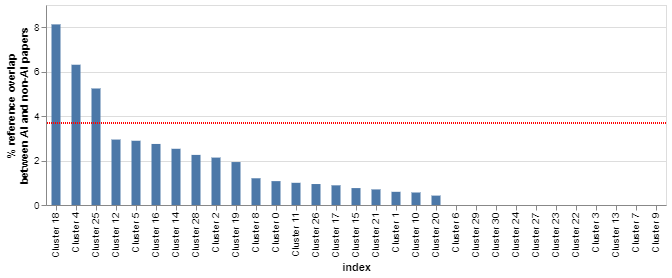
An interactive version of this graph is available in the online report
Findings – Quality
We conclude with an exploration of the quality of AI research to tackle COVID-19 and the levels of experience and track record of AI researchers who are reorienting their activities towards COVID-19, also checking the extent to which doing so involves large ‘jumps’ from the area where they worked before.
Here, it is important to note that we are using citation data as an imperfect proxy for the quality and influence of a publication. While we try to account for differences in citation rates across scholarly communities, and over time, by comparing citation levels inside topical clusters and circumscribing our analysis to 2020, we recognise the limitations of this measure of research quality and impact – expanding this with other indicators is an important issue for further research.
In Figure 11 we compare the distribution of citations across article sources, between COVID-19 and non-COVID-19 articles, and between AI and non-AI papers. The reason to distinguish between article sources is that these are used by researchers in communities in disciplines with different citation approaches.
The chart shows that most publications in the data have not received any citations, which is perhaps to be expected given that we are only focusing on papers published this year. In general, papers in biomedical sciences (bioRxiv) and medical sciences (medRxiv) tend to receive more citations, specially if they focus on COVID-19: the share of medRxiv COVID-19 papers with at least one citation is over five times higher than the share of non-COVID-19 papers from that source. The share of biorXiv papers about COVID-19 with at least one citation is almost four times higher. By contrast, the proportions of papers with at least one citation in arXiv is similar between COVID-19 and non-COVID-19 papers. When we compare COVID-19 and non-COVID-19 AI papers from different sources, we find that those in bioRxiv and medRxiv tend to have a higher share of papers with at least one citation, while AI papers about COVID-19 from arXiv tend to receive 14 per cent less citations than those that are not about COVID-19.
These differences could be explained by variation in the interest and quality of AI papers to tackle COVID-19 generated by different communities. In particular, one might expect AI applications developed by biotechnologists and medical scientists to involve a higher level of subject expertise than contributions coming from computer science, enhancing the latter’s ability to tackle COVID-19. It could also be that AI research to tackle COVID-19 published by computer scientists has less visibility (for example, as we noted in the introduction, arXiv is not even included in the CORD-19 dataset of scholarly work to tackle COVID-19).
Figure 11: COVID-19 papers (both AI and non-AI) tend to receive more citations in the medical and biological sciences, but not in arXiv
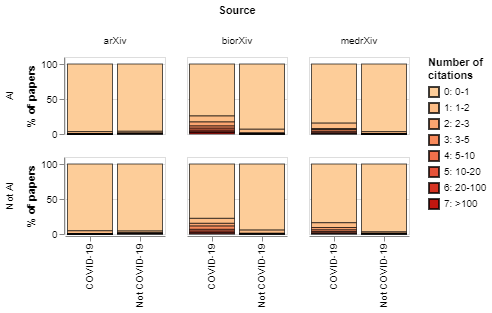
An interactive version of this graph is available in the online report
In figure 12 we compare the levels of citations across topical clusters and AI/non-AI categories. Each tick represents a paper, and the circles represent the number of citations received by papers at different levels. The charts are sorted in decreasing order of average citations. Clusters 12, 1 and 14, all of which relate to biomedical research, tend to receive the largest number of citations. Topical clusters dominated by AI such as cluster 16, cluster 19 and cluster 4 tend to receive less citations.
The citation distribution is highly skewed, with most papers receiving no citations at all and a handful of papers receiving hundreds of them. This makes it difficult to compare mean citation levels across groups. Having said this, we find that in only three of the top 12 topical clusters by levels of AI activity, the average citation counts for AI papers are higher than for non-AI papers (they are cluster 18, involving predictive analyses of hospital data, cluster 12, about vaccine discovery, and cluster 25, about COVID-19 diagnosis).
Figure 12: Number and frequency of citations for clusters with high levels of AI activity
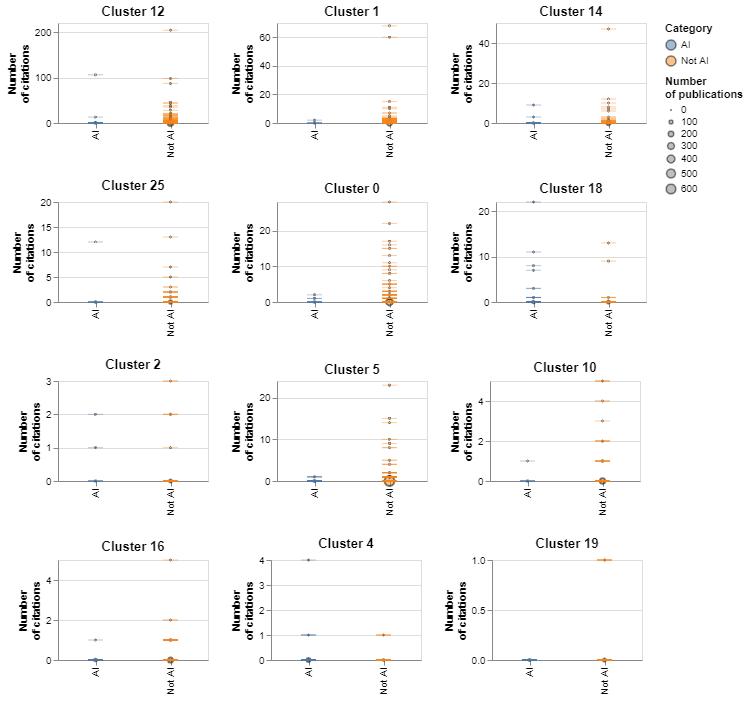
An interactive version of this graph is available in the online report
We have also studied the experience and track record of researchers participating in COVID-19 research. In order to do this, we look at the number of papers, and mean and median number of citations received by papers involving an author before 2020, focusing on recent publications (i.e. publications in 2018 or 2019). We then compare the means for those statistics between different groups of researchers, also testing whether any differences are statistically significant.[4]
In Figure 13 we compare our indicators of track record between researchers that have recently focused their work on COVID-19 and those that have not (first row), AI researchers that have focused on COVID-19 and those that have not (second row) and researchers tackling COVID-19 with or without AI (third row). We colour the bars based on the statistical significance of the results. The figure shows that:
- In general, researchers tackling COVID-19 have stronger track records: they have published more papers in recent years and those papers tend to receive more citations.
- When we focus on AI researchers, we find that researchers tackling COVID-19 tend to be more prolific and somewhat less likely to be cited (although some of the differences are not statistically significant).
- When we compare researchers tackling COVID-19 with AI with those using other techniques, we find that although AI researchers tend to be more prolific, they have a weaker track record, tending to have received less citations in previous work.
Figure 13: AI researchers tackling COVID-19 tend to have a less established track record (in terms of past citations) than non-AI researchers
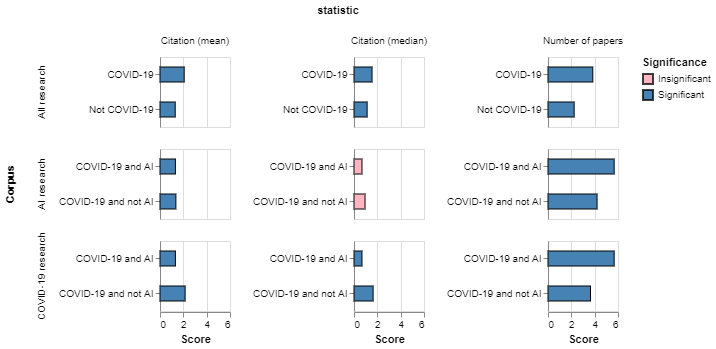
An interactive version of this graph is available in the online report
As before, there is the risk that some of the differences highlighted above may be driven by variation in citation propensities across research communities and disciplines, or varying levels of interest in research across domains. To account for this, in Figure 14 we compare the recent track record of AI and non-AI researchers active in the same topical cluster. We use the opacity of the bars to convey whether the differences being displayed are statistically significant or not.
We find that although researchers using AI to tackle COVID-19 tend to be somewhat more prolific (their mean number of publications is higher for 60 per cent of the clusters we consider), they are less established in their track records (in 90 per cent of the clusters non-AI researchers have higher citation medians, and in 80 per cent they have higher citation averages). All the statistically significant differences in citations are in favour of non-AI researchers, suggesting that they have authored more influential research in recent years.
Figure 14: AI researchers tend to have less established records when we compare them with non AI-researchers in the same topical cluster
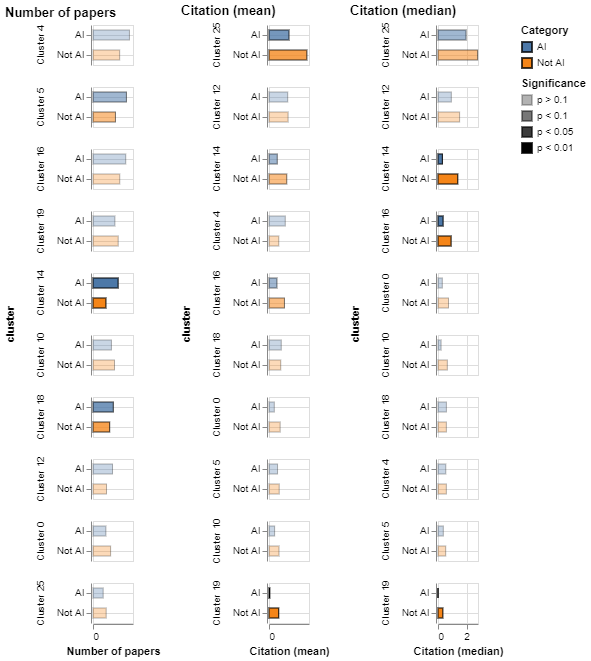
An interactive version of this graph is available in the online report
Lastly, we analyse the content of the contributions of AI researchers to examine their thematic diversity and how it changed due to publishing COVID-19-related work. In Figure 15 we show that COVID-19 publications increased the thematic diversity of most AI researchers. This increase is higher for those with less than 10 citations in total. We also find that COVID-19 publications increased the thematic diversity of AI researchers working in health while we identify a group of interdisciplinary researchers whose thematic diversity was already high and COVID-19 work did not alter it significantly. These findings are consistent with our citation analysis; researchers who were specialised on core AI and computer science topics made longer ‘jumps’ to contribute to COVID-19 than those with a cross-disciplinary profile.
Figure 15: Length of thematic jumps for AI researchers becoming active in COVID-19 research
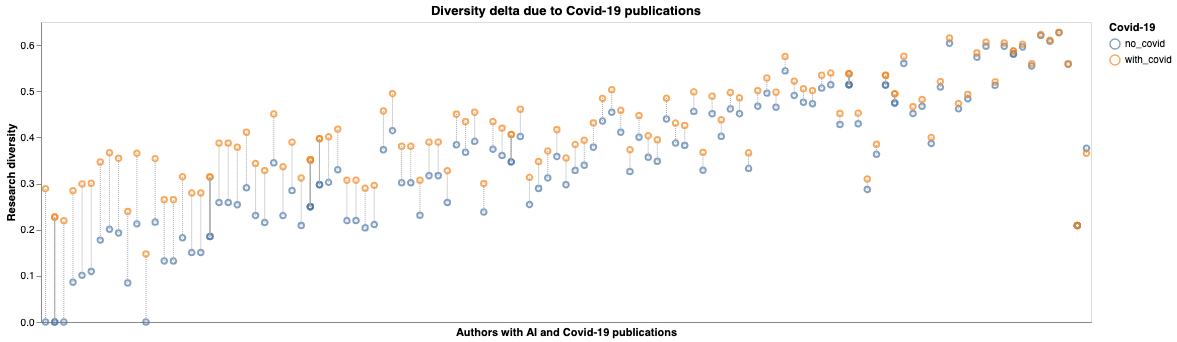
Footnotes
4. We do this using the Mann-Whitney test, which does not assume normality in the distribution of the statistics we are comparing.
Conclusion
We conclude by considering some implications of our findings. First, the persistent underrepresentation of artificial intelligence (AI) research in the COVID-19 mission field suggests some limitations in the generalisability of state-of-the-art algorithms into a new domain where data is fragmented, unreliable and sensitive, mistakes could cost lives and explainability is at a premium. Given this, and perhaps unsurprisingly, AI researchers have focused their efforts on computer vision and biomedical applications closer to their comfort zone at the risk of neglecting other important domains.
Second, we find some evidence of silos between AI researchers and those in medical and biological science disciplines in tackling the pandemic. AI researchers (and computer scientists) more broadly are sometimes accused of ‘solutionism’, looking for technological fixes for complex societal problems such as predicting and controlling the spread of a pandemic, ensuring the sustainability of public health systems or protecting the mental health of locked-down populations. It will be difficult for them to develop truly effective technologies to tackle these challenges without tapping on the knowledge of other disciplines, something that our analysis suggests is as common as it may be desired.
Third, there are concerns around quality. In recent years the AI research community has become increasingly concerned with low levels of reproducibility in AI research, as well as the prevalence of irresponsible modes of innovation where AI researchers ignore the repercussions and unintended consequences of the techniques that they develop. The comparatively low levels of citations received by AI researchers in our corpus, the weaker track record (in general) of AI researchers tackling COVID-19, the presence of a large number of research groups from unidentified institutions and the large thematic jumps from some researchers into COVID-19 field suggest that similar risks may be present in AI research oriented towards COVID-19. Researchers, policymakers and practitioners need to develop strategies to validate contributions from new entrants into the COVID-19 mission field, while ensuring that new voices and ideas can still be heard.
Of course, our analysis is not without limitations: we are focusing on COVID-19 research published in open preprints sites that may not be representative of wider research efforts to fight the pandemic. Going forward, it will be important to augment this analysis with similar studies of COVID-19 and AI publications in peer-reviewed sources. Our measures of quality and impact (citations) also have important limitations, not least heterogeneity in citation practices across disciplines and the risk of gaming. We have sought to address the first issue by comparing citation rates inside article sources and publication areas but more remains to be done – in particular, using other measures of influence (e.g. dissemination of research in social media) and impact (eg. identifying instances where research leads to patents, clinical trials, policy impacts and open source software applications). It is also worth noting that many of the methods that we have deployed in the paper, such as the topic modelling algorithms we use to segment COVID-19 research into topical clusters are experimental, so we advise caution in the interpretation of results. This is also why we are releasing all the code and data we have used in the paper, allowing other researchers to review, reproduce and build on our work.
Bibliography
Goodfellow, I., Bengio, Y., & Courville, A. (2016). Deep learning. MIT press.
Marcus, G. (2018). Deep learning: A critical appraisal. ArXiv Preprint ArXiv:1801.00631.
Mateos-Garcia, J. (Forthcoming). Mapping Research & Innovation Missions.
Mitchell, M. (2019). Artificial Intelligence: A Guide for Thinking Humans. Penguin UK.
Naudé, W. (2020a). Artificial Intelligence against COVID-19: An early review.
Sutton, R. S., & Barto, A. G. (2018). Reinforcement learning: An introduction. MIT press.
Wooldridge, M. (2020). The Road to Conscious Machines. Penguin Books, Limited.
Annex
List of terms similar to AI produced by word2vec:
['optimization_algorithm', 'convolutional_neural', 'automatic_detection', 'transfer_learning', 'semi_supervised', 'dnn', 'pre_trained', 'artificial_intelligence', 'natural_language', 'deep_learning', 'machine_learning', 'training_dataset', 'covid_net', 'classification', 'cnn', 'learning_based', 'deep_neural', 'neural_network', 'feature_extraction', 'deep_convolutional', 'supervised_learning', 'machine_learning_based', 'computational_intelligence', 'machine_intelligence']
Resources
Additional Resources
- COVID-19 Research Database (provided by the WHO)
- LitCOVID (provided by the NIH)
- COVID-19 Resource Page(provided by Microsoft)
- CORD-19 AI Powered Search (provided by Microsoft)
- COVID-19 Research Export File (provided by Dimensions)
- Day-Level COVID-19 Dataset (hosted on Kaggle)
- COVID-19 Global Cases (provided by Johns Hopkins University)
- COVID-19 Open Patent Dataset (hosted by Lens.org)
- COVID-19 Global Rheumatology Registry (provided by the Global Rheumatology Alliance)
- COVID-19 Literature Review Collection (hosted by Cochrane Library)
- CORD-19 Search Engine (provided by Verizon Media)
- COVID-19 Policy Dataset (provided by Overton)
- COVID-19 DOC Search Engine (provided by Doctor Evidence)
- Resource Page (provided by Doctor Evidence)
- COVID-19 Clinical Trials Tracker (provided by TranspariMED)
- ASReview - Active learning for Systematic Reviews (provided by Utrecht University)
- COVID-19 Public Media Dataset (provided by Anacode)
- CORD-19 Search (provided by AWS)
- COVID-19 Research Explorer (provided by Google)
- COVID-19 Intelligent Insight (provided by Sinequa)
- Blog Post: Computer Scientists Are Building Algorithms to Tackle COVID-19
- Visit CORD-19 Discourse for more resources
Publisher Resources
Compute Resources
AWS Resources and programs supporting COVID-19 research
- AWS Diagnostic Development Initiative Web Portal
- Registry of Open Data on AWS, COVID-19 Open Research Dataset (CORD-19)